Presented By: Interdisciplinary Seminar in Quantitative Methods (ISQM)
Interdisciplinary Seminar in Quantitative Methods (ISQM)
Accounting for Selection Bias due to Censoring by Death presented by Michael Elliott, University of Michigan
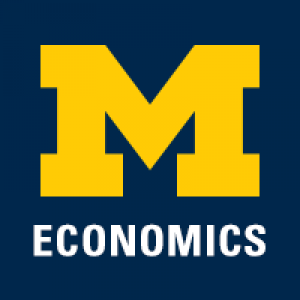
Abstract: Modern causal inference paradigms, with their emphasis on comparison of counterfactual outcomes, has led to increased appreciation of the potential for differential survival to bias estimates of effects on non-survival outcomes such as quality of life measures. Ignoring these selection effects might attenuate effect estimates, leading to settings in which exposures that shorten survival may appear to have little or no negative effects among observed survivors. For example, if those who experience rapid declines in wealth (“wealth shock”) later in life are both more likely to die at a younger age and have poorer health, the effect of wealth shock on self-reported health may be underestimated in the elderly.
In longitudinal studies, an increasingly common approach to deal with this selection issue is to estimate inverse probability weights (IPW) that “weight up” study persons remaining in the sample in proportion to their probability of death at a given wave of observation. This creates an “immortal” counterfactual population that, in many clinical and policy settings, is arguably not a sensible target of inference. A perhaps better alternative is to go the other direction and use the concept of principal stratification to target inference to the set of persons who would survive under all of the potential exposures under a comparison of interest (survivor average causal effects or SACEs). Recent work in marginal structural modeling has focused on developing methods to obtain unbiased SACE estimators by adapting marginal structural model weights to break the relationship between survival and exposure at each follow-up wave. I will discuss an alternative multiple imputation approach to generate counterfactual predictive distributions of principal strata to estimate SACEs. This talk will be primarily conceptual, and intended to spur discussion among the audience.
In longitudinal studies, an increasingly common approach to deal with this selection issue is to estimate inverse probability weights (IPW) that “weight up” study persons remaining in the sample in proportion to their probability of death at a given wave of observation. This creates an “immortal” counterfactual population that, in many clinical and policy settings, is arguably not a sensible target of inference. A perhaps better alternative is to go the other direction and use the concept of principal stratification to target inference to the set of persons who would survive under all of the potential exposures under a comparison of interest (survivor average causal effects or SACEs). Recent work in marginal structural modeling has focused on developing methods to obtain unbiased SACE estimators by adapting marginal structural model weights to break the relationship between survival and exposure at each follow-up wave. I will discuss an alternative multiple imputation approach to generate counterfactual predictive distributions of principal strata to estimate SACEs. This talk will be primarily conceptual, and intended to spur discussion among the audience.
Explore Similar Events
-
Loading Similar Events...