Presented By: ISR-Zwerdling Seminar in Labor Economics
Labor Economics
Human Decisions and Machine Predictions presented by Jens Ludwig, University of Chicago
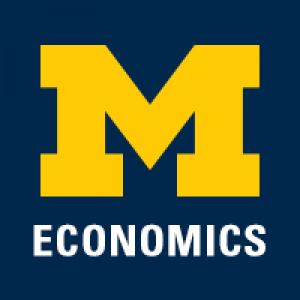
Abstract:
Each year judges across the United States make millions of decisions about whether someone
who has just been arrested should be set free or detained in jail as they await resolution of their
cases. Besides its importance, this problem is interesting because it is a canonical case where
empirical work can inform policy not through causal analysis, but -- because the jail decision is
by law supposed to focus just on the defendant's risk to public safety or of flight - through prediction. Machine learning tools provide a way to analyze these prediction policy problems since -- unlike standard econometric tools -- they are designed to optimize prediction accuracy. We compare how these tools fare relative to judges on a national dataset on over 150,000 felony cases. We find that the release rule based on machine learning predictions would enable us to reduce the jail population without any increase in the crime rate, or let us reduce crime rates without changing the jail population. These gains are larger than those obtained from using standard logistic regression. One potential problem in evaluating performance of these predictions is that we only observe behavior (``labels'') for released defendants. We describe a technique for solving this problem and argue this
selective labels problem does not lead to much bias in our particular application. To understand why judges' predictions may be inaccurate, we use a separate machine learning algorithm to predict the judges' release decisions. Even though this algorithm does not have access to crime data, we find that the predicted judge outperforms the judge, suggesting judges misuse "unobservables" such as the defendant's appearance or demeanor in court.
Each year judges across the United States make millions of decisions about whether someone
who has just been arrested should be set free or detained in jail as they await resolution of their
cases. Besides its importance, this problem is interesting because it is a canonical case where
empirical work can inform policy not through causal analysis, but -- because the jail decision is
by law supposed to focus just on the defendant's risk to public safety or of flight - through prediction. Machine learning tools provide a way to analyze these prediction policy problems since -- unlike standard econometric tools -- they are designed to optimize prediction accuracy. We compare how these tools fare relative to judges on a national dataset on over 150,000 felony cases. We find that the release rule based on machine learning predictions would enable us to reduce the jail population without any increase in the crime rate, or let us reduce crime rates without changing the jail population. These gains are larger than those obtained from using standard logistic regression. One potential problem in evaluating performance of these predictions is that we only observe behavior (``labels'') for released defendants. We describe a technique for solving this problem and argue this
selective labels problem does not lead to much bias in our particular application. To understand why judges' predictions may be inaccurate, we use a separate machine learning algorithm to predict the judges' release decisions. Even though this algorithm does not have access to crime data, we find that the predicted judge outperforms the judge, suggesting judges misuse "unobservables" such as the defendant's appearance or demeanor in court.
Explore Similar Events
-
Loading Similar Events...