Presented By: Department of Statistics
Dissertation Defense: Topics in Network Analysis with Applications to Brain Connectomics
Jesús Arroyo
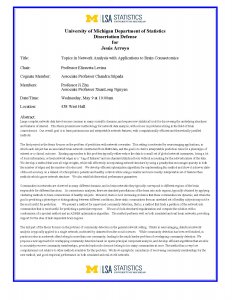
Large complex network data have become common in many scientific domains, and require new statistical tools for discovering the underlying structures and features of interest. This thesis presents new methodology for network data analysis, with a focus on problems arising in the field of brain connectomics. Our overall goal is to learn parsimonious and interpretable network features, with computationally efficient and theoretically justified methods.
The first project in the thesis focuses on the problem of prediction with network covariates. This setting is motivated by neuroimaging applications, in which each subject has an associated brain network constructed from fMRI data, and the goal is to derive interpretable prediction rules for a phenotype of interest or a clinical outcome. Existing approaches to this problem typically either reduce the data to a small set of global network summaries, losing a lot of local information, or treat network edges as a ``bag of features'' and use standard statistical tools without accounting for the network nature of the data. We develop a method that uses all edge weights, while still effectively incorporating network structure by using a penalty that encourages sparsity in both the number of edges and the number of nodes used. We develop efficient optimization algorithms for implementing this method and show it achieves state-of-the-art accuracy on a dataset of schizophrenic patients and healthy controls while using a smaller and more readily interpretable set of features than methods which ignore network structure. We also establish theoretical performance guarantees.
Communities in networks are observed in many different domains, and in brain networks they typically correspond to different regions of the brain responsible for different functions. In connectomic analyses, there are standard parcellations of the brain into such regions, typically obtained by applying clustering methods to brain connectomes of healthy subjects. However, there is now increasing evidence that these communities are dynamic, and when the goal is predicting a phenotype or distinguishing between different conditions, these static communities from an unrelated set of healthy subjects may not be the most useful for prediction. We present a method for supervised community detection, that is, a method that finds a partition of the network into communities that is most useful for predicting a particular response. We use a block-structured regularization and compute the solution with a combination of a spectral method and an ADMM optimization algorithm. The method performs well on both simulated and real brain networks, providing support for the idea of task-dependent brain regions.
The last part of the thesis focuses on the problem of community detection in the general network setting. Unlike in neuroimaging, statistical network analysis is typically applied to a single network, motivated by datasets from the social sciences. While community detection has been well studied, in practice nodes in a network often belong to more than one community, leading to the much harder problem of overlapping community detection. We propose a new approach for overlapping community detection based on sparse principal component analysis, and develop efficient algorithms that are able to accurately recover community memberships, provided each node does not belong to too many communities at once. The method has a very low computational cost relative to other methods available for this problem. We show asymptotic consistency of recovering community memberships by the new method, and good empirical performance on both simulated and real-world networks.
The first project in the thesis focuses on the problem of prediction with network covariates. This setting is motivated by neuroimaging applications, in which each subject has an associated brain network constructed from fMRI data, and the goal is to derive interpretable prediction rules for a phenotype of interest or a clinical outcome. Existing approaches to this problem typically either reduce the data to a small set of global network summaries, losing a lot of local information, or treat network edges as a ``bag of features'' and use standard statistical tools without accounting for the network nature of the data. We develop a method that uses all edge weights, while still effectively incorporating network structure by using a penalty that encourages sparsity in both the number of edges and the number of nodes used. We develop efficient optimization algorithms for implementing this method and show it achieves state-of-the-art accuracy on a dataset of schizophrenic patients and healthy controls while using a smaller and more readily interpretable set of features than methods which ignore network structure. We also establish theoretical performance guarantees.
Communities in networks are observed in many different domains, and in brain networks they typically correspond to different regions of the brain responsible for different functions. In connectomic analyses, there are standard parcellations of the brain into such regions, typically obtained by applying clustering methods to brain connectomes of healthy subjects. However, there is now increasing evidence that these communities are dynamic, and when the goal is predicting a phenotype or distinguishing between different conditions, these static communities from an unrelated set of healthy subjects may not be the most useful for prediction. We present a method for supervised community detection, that is, a method that finds a partition of the network into communities that is most useful for predicting a particular response. We use a block-structured regularization and compute the solution with a combination of a spectral method and an ADMM optimization algorithm. The method performs well on both simulated and real brain networks, providing support for the idea of task-dependent brain regions.
The last part of the thesis focuses on the problem of community detection in the general network setting. Unlike in neuroimaging, statistical network analysis is typically applied to a single network, motivated by datasets from the social sciences. While community detection has been well studied, in practice nodes in a network often belong to more than one community, leading to the much harder problem of overlapping community detection. We propose a new approach for overlapping community detection based on sparse principal component analysis, and develop efficient algorithms that are able to accurately recover community memberships, provided each node does not belong to too many communities at once. The method has a very low computational cost relative to other methods available for this problem. We show asymptotic consistency of recovering community memberships by the new method, and good empirical performance on both simulated and real-world networks.
Explore Similar Events
-
Loading Similar Events...