Presented By: Biomedical Engineering
Ph.D. Defense: Dakota Crisp
Evaluating a Taxonomy of Seizure Dynamics
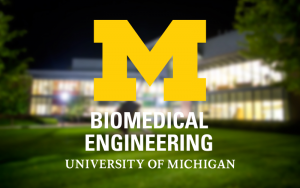
Epilepsy is characterized by spontaneously recurring seizures that severely disrupt quality of life and pose risks of injury and death. It is a highly heterogenous disease, with seizures seen in a wide range of other diseases (Alzheimer’s, autism, Down’s syndrome, etc.). Yet to date, there is no method of categorizing seizures that can help distinguish the link between pathology and seizures. The aim of this work is to validate and explore a method of categorizing seizures based on their fundamental dynamics to provide a framework for future research to better investigate the underlying mechanisms of seizures and therapeutic approaches to stop them.
The first study used predictions from a previously-published computational model to visually classify seizures using dynamical transition features in two large datasets (simulated and real human data). Machine learning was applied to raw signal features to verify the accuracy of the reviewer’s labels. It found that visual classification is consistent and supported by the signal feature analysis. We also investigate the model’s predictions in real human data, finding that most dynamic classifications were observed and patients can have varying seizure dynamics over time. A major unpublished aspect of this work is that the human data analysis was crucial in the original development of the model.
The second study used data mining and machine learning in a long-term rat model of epileptogenesis to investigate the viability of these same dynamic principles as a biomarker of epileptic brain state. It also applied the same rigor to an analysis of the response to electrical stimulation. We found that evoked responses can be used to predict if an injured brain would eventually develop seizures or not. Once seizures began manifesting, both evoked responses and seizure onset dynamics had strong correlation with the progression of epileptogenesis, suggesting they are independent biomarkers.
For the final study, we use the same principles of dynamics and machine learning to characterize differences between a low Mg2+ / high K+ mouse brain-slice seizure model with and without different anti-seizure drugs. It found that anti-seizure drugs can change the observed seizure dynamics, and each drug has a different effect on brain dynamics.
These three studies provide evidence that seizures can be categorized by their fundamental dynamics. These dynamics can provide mechanistic insights into current brain state, future brain states, and the response to anti-epileptic drugs. The results presented in this dissertation can be used as a framework to further investigate seizure mechanisms and personalize patient treatment and research.
The first study used predictions from a previously-published computational model to visually classify seizures using dynamical transition features in two large datasets (simulated and real human data). Machine learning was applied to raw signal features to verify the accuracy of the reviewer’s labels. It found that visual classification is consistent and supported by the signal feature analysis. We also investigate the model’s predictions in real human data, finding that most dynamic classifications were observed and patients can have varying seizure dynamics over time. A major unpublished aspect of this work is that the human data analysis was crucial in the original development of the model.
The second study used data mining and machine learning in a long-term rat model of epileptogenesis to investigate the viability of these same dynamic principles as a biomarker of epileptic brain state. It also applied the same rigor to an analysis of the response to electrical stimulation. We found that evoked responses can be used to predict if an injured brain would eventually develop seizures or not. Once seizures began manifesting, both evoked responses and seizure onset dynamics had strong correlation with the progression of epileptogenesis, suggesting they are independent biomarkers.
For the final study, we use the same principles of dynamics and machine learning to characterize differences between a low Mg2+ / high K+ mouse brain-slice seizure model with and without different anti-seizure drugs. It found that anti-seizure drugs can change the observed seizure dynamics, and each drug has a different effect on brain dynamics.
These three studies provide evidence that seizures can be categorized by their fundamental dynamics. These dynamics can provide mechanistic insights into current brain state, future brain states, and the response to anti-epileptic drugs. The results presented in this dissertation can be used as a framework to further investigate seizure mechanisms and personalize patient treatment and research.
Explore Similar Events
-
Loading Similar Events...