Presented By: Michigan Robotics
Collection and Analysis of Driving Videos based on Traffic Participants
Robotics PhD Defense, Brian Yao
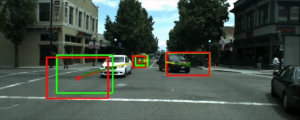
Autonomous vehicle (AV) prototypes have been deployed in increasingly varied environments in recent years. An AV must be able to reliably detect and predict the future motion of traffic participants to maintain safe operation based on data collected from high-quality onboard sensors. Sensors such as camera and LiDAR generate high-bandwidth data that requires substantial computational and memory resources. To address these AV challenges, this thesis investigates three related problems: 1) What will the observed traffic participants do? 2) Is an anomalous traffic event likely to happen in near future? and 3) How should we collect fleet-wide high-bandwidth data based on 1) and 2) over the long-term?
The first problem is addressed with future traffic trajectory and pedestrian behavior prediction.We propose a future object localization (FOL) method for trajectory prediction in first person videos (FPV). FOL encodes heterogeneous observations including bounding boxes, optical flow features and ego camera motions with multi-stream recurrent neural networks (RNN) to predict future trajectories. We then introduce BiTraP, a goal-conditioned bidirectional multi-modal trajectory prediction method. BiTraP estimates multi-modal trajectories and uses novel bi-directional decoder and loss to improve longer-term trajectory prediction accuracy. We show that different choices of non-parametric versus parametric target models directly influence predicted multi-modal trajectory distributions. Experiments with two FPV and six bird's-eye view (BEV) datasets show the effectiveness of our methods compared to state-of-the-art. We define pedestrian behavior prediction as a combination of action and intent. We hypothesize that current and future actions are strong intent priors and propose a multi-task leaning RNN encoder-decoder network to detect and predict future pedestrian actions and street crossing intent. Experimental results show that one task helps the other so they together achieve state-of-the-art performance on published datasets.
To identify likely traffic anomaly events, we propose to predict locations of traffic participants over a near-term future horizon and monitor accuracy and consistency of these predictions as evidence of an anomaly. Inconsistent predictions tend to indicate an anomaly has or is about to occur. A supervised video action recognition method can then be applied to classify detected anomalies. We introduce a spatial-temporal area under curve (STAUC) metric as a supplement to the existing area under curve (AUC) evaluation and show it captures how well a model detects both temporal and spatial locations of anomalous events. Experimental results show the proposed method and consistency-based anomaly score are more robust to moving cameras than image generation based methods; our method achieves state-of-the-art performance over AUC and STAUC metrics.
Video anomaly detection (VAD) and action recognition support event-of-interest (EOI) distinction from normal driving data. We introduce a Smart Black Box (SBB), an intelligent event data recorder, to prioritize EOI data in long-term driving. The SBB compresses high-bandwidth data based on EOI potential and on-board storage limits. The SBB is designed to prioritize newer and anomalous driving data and discard older and normal data. An optimal compression factor is selected based on the trade-off between data value and storage cost.Experiments in a traffic simulator and with real-world datasets show the efficiency and effectiveness of using a SBB to collect high-quality videos in long-term driving.
The first problem is addressed with future traffic trajectory and pedestrian behavior prediction.We propose a future object localization (FOL) method for trajectory prediction in first person videos (FPV). FOL encodes heterogeneous observations including bounding boxes, optical flow features and ego camera motions with multi-stream recurrent neural networks (RNN) to predict future trajectories. We then introduce BiTraP, a goal-conditioned bidirectional multi-modal trajectory prediction method. BiTraP estimates multi-modal trajectories and uses novel bi-directional decoder and loss to improve longer-term trajectory prediction accuracy. We show that different choices of non-parametric versus parametric target models directly influence predicted multi-modal trajectory distributions. Experiments with two FPV and six bird's-eye view (BEV) datasets show the effectiveness of our methods compared to state-of-the-art. We define pedestrian behavior prediction as a combination of action and intent. We hypothesize that current and future actions are strong intent priors and propose a multi-task leaning RNN encoder-decoder network to detect and predict future pedestrian actions and street crossing intent. Experimental results show that one task helps the other so they together achieve state-of-the-art performance on published datasets.
To identify likely traffic anomaly events, we propose to predict locations of traffic participants over a near-term future horizon and monitor accuracy and consistency of these predictions as evidence of an anomaly. Inconsistent predictions tend to indicate an anomaly has or is about to occur. A supervised video action recognition method can then be applied to classify detected anomalies. We introduce a spatial-temporal area under curve (STAUC) metric as a supplement to the existing area under curve (AUC) evaluation and show it captures how well a model detects both temporal and spatial locations of anomalous events. Experimental results show the proposed method and consistency-based anomaly score are more robust to moving cameras than image generation based methods; our method achieves state-of-the-art performance over AUC and STAUC metrics.
Video anomaly detection (VAD) and action recognition support event-of-interest (EOI) distinction from normal driving data. We introduce a Smart Black Box (SBB), an intelligent event data recorder, to prioritize EOI data in long-term driving. The SBB compresses high-bandwidth data based on EOI potential and on-board storage limits. The SBB is designed to prioritize newer and anomalous driving data and discard older and normal data. An optimal compression factor is selected based on the trade-off between data value and storage cost.Experiments in a traffic simulator and with real-world datasets show the efficiency and effectiveness of using a SBB to collect high-quality videos in long-term driving.
Livestream Information
ZoomDecember 3, 2020 (Thursday) 4:00pm
Meeting ID: 99967072867
Explore Similar Events
-
Loading Similar Events...