Presented By: U-M Industrial & Operations Engineering
PhD Researach Talk: Seokhyun Chung
Collaborative data analytics for smart & connected systems
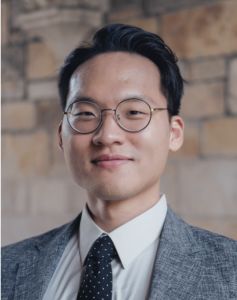
Revolutionary advances in Internet of Things (IoT) technologies have realized the emergence of smart & connected systems, where physical units (or devices) that acquire data are connected through a central cloud with intelligence. Increased connectivity across units provides significant opportunities for improving smart analytics through sharing knowledge across units, but, at the same time, reveals critical challenges. First, skyrocketing data scale is beginning to overwhelm computing, storage, and bandwidth resources in the central cloud. Second, personalized analytics is indispensable as units often operate in different environments or have unique features. Third, real-time data collection in smart & connected systems necessitates adapting a smart analytics model to the new data collected.
In this talk, I will present two approaches that address the challenges above, based on a collaborative strategy that distributes learning efforts to the units at the edge while keeping their data stored locally. First, I will discuss a method where physical units collaboratively estimate a multi-output Gaussian process model by exploiting the natural hierarchy of smart & connected systems. Next, I will discuss a two-step approach that (i) finds a well-generalized solution lying in flat minima on the loss surface through distributed computation across units and then (ii) personalizes the learned model to new units without losing old knowledge. Real-world applications for the prediction of lithium-ion battery degradation, condition monitoring signals of turbofan engines, and missing climate data highlight the advantageous features of the proposed models. The talk concludes with some interesting future directions.
Presenter Bio:
Seokhyun Chung is a Ph.D. candidate in the Department of Industrial & Operations Engineering at the University of Michigan, where he is advised by Dr. Raed Al Kontar. His research interests broadly lie in data-driven predictive analytics and decision-making for smart & connected systems. His current research explores collaborative and distributed analytics where Internet of Things-enabled entities (e.g., smartphones, electric vehicles, and wearable devices) exploit their edge computing power to build smart analytics collaboratively. He has been nominated as a finalist in the Quality, Statistics, & Reliability (QSR) Best refereed paper competition at INFORMS 2022 and the Quality Control & Reliability Engineering (QCRE) Best student paper competition at IISE 2021.
In this talk, I will present two approaches that address the challenges above, based on a collaborative strategy that distributes learning efforts to the units at the edge while keeping their data stored locally. First, I will discuss a method where physical units collaboratively estimate a multi-output Gaussian process model by exploiting the natural hierarchy of smart & connected systems. Next, I will discuss a two-step approach that (i) finds a well-generalized solution lying in flat minima on the loss surface through distributed computation across units and then (ii) personalizes the learned model to new units without losing old knowledge. Real-world applications for the prediction of lithium-ion battery degradation, condition monitoring signals of turbofan engines, and missing climate data highlight the advantageous features of the proposed models. The talk concludes with some interesting future directions.
Presenter Bio:
Seokhyun Chung is a Ph.D. candidate in the Department of Industrial & Operations Engineering at the University of Michigan, where he is advised by Dr. Raed Al Kontar. His research interests broadly lie in data-driven predictive analytics and decision-making for smart & connected systems. His current research explores collaborative and distributed analytics where Internet of Things-enabled entities (e.g., smartphones, electric vehicles, and wearable devices) exploit their edge computing power to build smart analytics collaboratively. He has been nominated as a finalist in the Quality, Statistics, & Reliability (QSR) Best refereed paper competition at INFORMS 2022 and the Quality Control & Reliability Engineering (QCRE) Best student paper competition at IISE 2021.
Explore Similar Events
-
Loading Similar Events...