Presented By: Gender Diversity in Robotics
GENDiR Seminar Series: Transfer Learning for Robots and for Humans
Tabitha Edith Lee
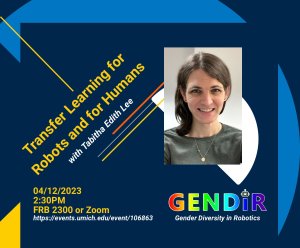
Abstract:
Transfer learning --- how information that is learned in one context can be transferred for use in a different setting --- has far-reaching consequences for agents operating under domain shifts. This talk will present lessons learned in transfer learning for robots and for humans. First, the methodology of structural sim-to-real transfer for robots will be presented, which aims to learn the causal structure of a robot manipulation policy or skill in simulation first before deployment on a real robot system. This section of the talk will summarize two algorithms in this area, CREST and SCALE, that were recognized by NCWIT as a Collegiate Award Honorable Mention. I will then draw parallels to how transfer learning can empower navigation of domain shifts in humans using my gender and physical disability transitions as examples. This section will identify human attributes and characteristics, such as empathy, compassion, understanding, and appreciation of differences, that bolster other humans experiencing life transitions. Lastly, I will summarize work done towards teaching such attributes for first-year Ph.D. students at Carnegie Mellon University through the development and launch of 15-996: Introduction to Justice, Equity, Diversity and Inclusion in Computer Science.
Speaker Bio:
Tabitha Edith Lee is a fifth-year Ph.D. candidate in Robotics at Carnegie Mellon University’s Robotics Institute. She is a member of the Intelligent Autonomous Manipulation Lab and advised by Prof. Oliver Kroemer. Her thesis research investigates causal robot learning for manipulation: the interplay between robot perception and control through the lens of causality to learn and leverage the causal structure of manipulation tasks. Her research in structural sim-to-real transfer has been recognized by an Honorable Mention selection for the NCWIT Collegiate Award. She is also a Siebel Scholar in Computer Science.
Transfer learning --- how information that is learned in one context can be transferred for use in a different setting --- has far-reaching consequences for agents operating under domain shifts. This talk will present lessons learned in transfer learning for robots and for humans. First, the methodology of structural sim-to-real transfer for robots will be presented, which aims to learn the causal structure of a robot manipulation policy or skill in simulation first before deployment on a real robot system. This section of the talk will summarize two algorithms in this area, CREST and SCALE, that were recognized by NCWIT as a Collegiate Award Honorable Mention. I will then draw parallels to how transfer learning can empower navigation of domain shifts in humans using my gender and physical disability transitions as examples. This section will identify human attributes and characteristics, such as empathy, compassion, understanding, and appreciation of differences, that bolster other humans experiencing life transitions. Lastly, I will summarize work done towards teaching such attributes for first-year Ph.D. students at Carnegie Mellon University through the development and launch of 15-996: Introduction to Justice, Equity, Diversity and Inclusion in Computer Science.
Speaker Bio:
Tabitha Edith Lee is a fifth-year Ph.D. candidate in Robotics at Carnegie Mellon University’s Robotics Institute. She is a member of the Intelligent Autonomous Manipulation Lab and advised by Prof. Oliver Kroemer. Her thesis research investigates causal robot learning for manipulation: the interplay between robot perception and control through the lens of causality to learn and leverage the causal structure of manipulation tasks. Her research in structural sim-to-real transfer has been recognized by an Honorable Mention selection for the NCWIT Collegiate Award. She is also a Siebel Scholar in Computer Science.
Livestream Information
ZoomApril 12, 2023 (Wednesday) 2:30pm
Meeting ID: 93957867552
Meeting Password: gendir
Explore Similar Events
-
Loading Similar Events...