Presented By: Biomedical Engineering
Hallucinations and objective assessments of deep learning technologies for medical image formation
Biomedical Engineering (BME 500) Seminar Series - Mark Anastasio, PhD
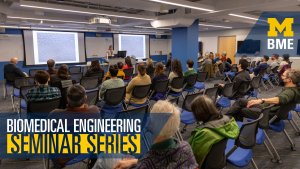
Abstract:
A variety of deep learning-based image restoration and reconstruction methods, generically referred to as image formation methods, have been proposed for use with biomedical images. It is widely accepted that the assessment and refinement of biomedical imaging technologies should be performed by objective, i.e., task-based, measures of image quality (IQ). However, the objective evaluation of deep learning-based image formation technologies remains largely lacking, despite the breakneck speed at which they are being developed. As such, there is an ever-growing collection of methods whose utility and trustworthiness remains largely unknown. Moreover, such methods have the capability to ‘hallucinate’ false structures, which is of significant concern in medical imaging applications. In this work, we report studies in which the performance of deep learning-based image restoration methods is objectively assessed. The performance of the ideal observer (IO) and common linear numerical observers are quantified, and detection efficiencies are computed to assess the impact of deep learning image formation methods on signal detection performance. The numerical results indicate that, in the cases considered, the application of a deep image formation network can result in a loss of task-relevant information in the image, despite improvement in traditional computer-vision metrics. We also demonstrate that traditional and objective IQ measures can vary in opposite ways as a function of network depth. These results highlight the need for the objective evaluation of IQ for deep image formation technologies and may suggest future avenues for improving the effectiveness of medical imaging applications.
Bio:
Dr. Mark Anastasio is the Donald Biggar Willett Professor in Engineering and the Head of the Department of Bioengineering at the University of Illinois at Urbana-Champaign (UIUC). Before joining UIUC in 2019, he was a Professor of Biomedical Engineering at Washington University in St. Louis, where he established one of the nation’s first stand-alone PhD programs in imaging science. Dr. Anastasio’s research accomplishments to the fields of biomedical imaging and image science have been numerous and impactful and his general interests broadly address the computational aspects of image formation, modern imaging science, and applied machine learning. He has conducted research in the fields of diffraction tomography, X-ray phase-contrast imaging, and ultrasound tomography. He one of the world’s leading authorities on photoacoustic computed tomography (PACT) and has made numerous and important contributions to development of PACT for over fifteen years. He has published over 175 peer-reviewed journal papers in leading imaging and optical science journals and was the recipient of a National Science Foundation (NSF) CAREER Award to develop image reconstruction methods. He is a Fellow of the American Institute for Medical and Biological Engineering (AIMBE), the International Academy of Medical and Biological Engineering (IAMBE) and the SPIE. He also served as the Chair of the NIH BMIT-B and EITA Study Sections.
Zoom:
https://umich.zoom.us/j/91712262512
A variety of deep learning-based image restoration and reconstruction methods, generically referred to as image formation methods, have been proposed for use with biomedical images. It is widely accepted that the assessment and refinement of biomedical imaging technologies should be performed by objective, i.e., task-based, measures of image quality (IQ). However, the objective evaluation of deep learning-based image formation technologies remains largely lacking, despite the breakneck speed at which they are being developed. As such, there is an ever-growing collection of methods whose utility and trustworthiness remains largely unknown. Moreover, such methods have the capability to ‘hallucinate’ false structures, which is of significant concern in medical imaging applications. In this work, we report studies in which the performance of deep learning-based image restoration methods is objectively assessed. The performance of the ideal observer (IO) and common linear numerical observers are quantified, and detection efficiencies are computed to assess the impact of deep learning image formation methods on signal detection performance. The numerical results indicate that, in the cases considered, the application of a deep image formation network can result in a loss of task-relevant information in the image, despite improvement in traditional computer-vision metrics. We also demonstrate that traditional and objective IQ measures can vary in opposite ways as a function of network depth. These results highlight the need for the objective evaluation of IQ for deep image formation technologies and may suggest future avenues for improving the effectiveness of medical imaging applications.
Bio:
Dr. Mark Anastasio is the Donald Biggar Willett Professor in Engineering and the Head of the Department of Bioengineering at the University of Illinois at Urbana-Champaign (UIUC). Before joining UIUC in 2019, he was a Professor of Biomedical Engineering at Washington University in St. Louis, where he established one of the nation’s first stand-alone PhD programs in imaging science. Dr. Anastasio’s research accomplishments to the fields of biomedical imaging and image science have been numerous and impactful and his general interests broadly address the computational aspects of image formation, modern imaging science, and applied machine learning. He has conducted research in the fields of diffraction tomography, X-ray phase-contrast imaging, and ultrasound tomography. He one of the world’s leading authorities on photoacoustic computed tomography (PACT) and has made numerous and important contributions to development of PACT for over fifteen years. He has published over 175 peer-reviewed journal papers in leading imaging and optical science journals and was the recipient of a National Science Foundation (NSF) CAREER Award to develop image reconstruction methods. He is a Fellow of the American Institute for Medical and Biological Engineering (AIMBE), the International Academy of Medical and Biological Engineering (IAMBE) and the SPIE. He also served as the Chair of the NIH BMIT-B and EITA Study Sections.
Zoom:
https://umich.zoom.us/j/91712262512
Livestream Information
ZoomApril 6, 2023 (Thursday) 3:30pm
Meeting ID: 91712262512
Explore Similar Events
-
Loading Similar Events...