Presented By: Ecology and Evolutionary Biology
EEB Tuesday Lunch Seminar - Unsupervised Learning as an Analog for Evolvability in Genotype-Phenotype Maps
with Matthew Moreno, EEB Postdoctoral Fellow, Zaman Lab
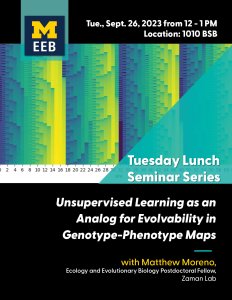
This event is part of Ecology and Evolutionary Biology's weekly lunch series.
About: The capability of an evolutionary substrate to generate novel phenotypic variation that is viable under mutation, referred to as evolvability, underpins the process of adaptive evolution. However, evolutionary simulations using models with high-dimensional phenotypes often exhibit stunted evolvability in the absence of indirect genotype-phenotype mapping that facilitates coordinated changes over many phenotypic traits. If these genotype-phenotype maps bias toward phenotypic viability and maintain phenotypic diversity, the resulting genetic search space will be lower-dimensional and less rugged, making it more conducive to adaptive evolution. Such evolvability in genotype-phenotype maps shares significant conceptual overlap with unsupervised learning, which extracts regularities and structure from unlabeled data that can enable lower-dimensional, compact representations of complex data like images and text. Here, we report a suite of benchmark fitness landscapes designed to facilitate head-to-head comparison of unsupervised learning techniques and evolved genotype-phenotype maps. This framework will contribute critical experimental rigor to ongoing efforts to harness unsupervised learning as a theoretical framework to understand evolvability. Exploration of unsupervised learning methods in engineering evolvable genotype-phenotype maps has great promise to benefit application-oriented evolutionary computation, as well.
About: The capability of an evolutionary substrate to generate novel phenotypic variation that is viable under mutation, referred to as evolvability, underpins the process of adaptive evolution. However, evolutionary simulations using models with high-dimensional phenotypes often exhibit stunted evolvability in the absence of indirect genotype-phenotype mapping that facilitates coordinated changes over many phenotypic traits. If these genotype-phenotype maps bias toward phenotypic viability and maintain phenotypic diversity, the resulting genetic search space will be lower-dimensional and less rugged, making it more conducive to adaptive evolution. Such evolvability in genotype-phenotype maps shares significant conceptual overlap with unsupervised learning, which extracts regularities and structure from unlabeled data that can enable lower-dimensional, compact representations of complex data like images and text. Here, we report a suite of benchmark fitness landscapes designed to facilitate head-to-head comparison of unsupervised learning techniques and evolved genotype-phenotype maps. This framework will contribute critical experimental rigor to ongoing efforts to harness unsupervised learning as a theoretical framework to understand evolvability. Exploration of unsupervised learning methods in engineering evolvable genotype-phenotype maps has great promise to benefit application-oriented evolutionary computation, as well.