Presented By: Michigan Robotics
Spatial-Semantic 3D Robot Perception with Computational Symmetries
PhD Defense, Ray Zhang
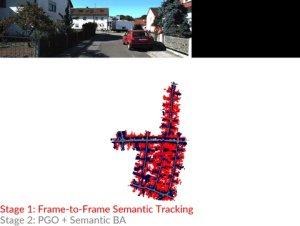
Co-Chairs: Maani Ghaffari and Ryan Eustice
Robot localization and mapping is a process where a robot constructs a global spatial model of a scene based on multiple local observations. The primary challenges involve the high degree of noise and outliers within sensor observations, and the integration of semantic information into actionable insights. In this presentation, we utilize the tools from the reproducing kernel Hilbert space and the equivariance learning theory, considering the symmetry of input data. These enable us to formulate two-frame point cloud registration and multi-frame bundle adjustment algorithms that demonstrate robustness against input noise and outliers. Additionally, this framework can tightly interweave spatial-semantic relationships, subsequently augmenting various downstream applications like mapping and exploration.
Zoom password: rzh
Robot localization and mapping is a process where a robot constructs a global spatial model of a scene based on multiple local observations. The primary challenges involve the high degree of noise and outliers within sensor observations, and the integration of semantic information into actionable insights. In this presentation, we utilize the tools from the reproducing kernel Hilbert space and the equivariance learning theory, considering the symmetry of input data. These enable us to formulate two-frame point cloud registration and multi-frame bundle adjustment algorithms that demonstrate robustness against input noise and outliers. Additionally, this framework can tightly interweave spatial-semantic relationships, subsequently augmenting various downstream applications like mapping and exploration.
Zoom password: rzh