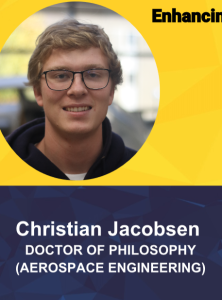
Despite the potential of Machine learning (ML) to enhance scientific discovery
and engineering innovation, applications of ML in physical problems often
grapple with a critical challenge: predictions frequently lack transparency and
interpretability, ultimately compromising generalizability and hindering the
diagnosis of limitations. This work presents approaches that use ML to learn
from data while increasing the interpretability of models, leading to more
generalizable, reliable, and safe physical models that improve accuracy and/or
computational efficiency over traditional methods.
and engineering innovation, applications of ML in physical problems often
grapple with a critical challenge: predictions frequently lack transparency and
interpretability, ultimately compromising generalizability and hindering the
diagnosis of limitations. This work presents approaches that use ML to learn
from data while increasing the interpretability of models, leading to more
generalizable, reliable, and safe physical models that improve accuracy and/or
computational efficiency over traditional methods.
Livestream Information
LivestreamMarch 19, 2024 (Tuesday) 10:30am
Meeting Password: 019328
Explore Similar Events
-
Loading Similar Events...