Presented By: Industrial & Operations Engineering
899 Seminar Series: Ashwin Pananjady
Sharply predicting the behavior of complex iterative algorithms with random data
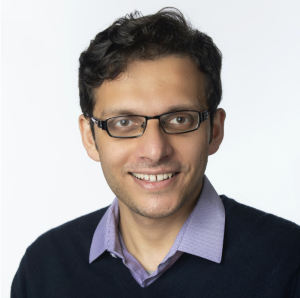
Presenter Bio:
Ashwin Pananjady is an Assistant Professor at Georgia Tech, with a joint appointment between the Schools of Industrial and Systems Engineering and Electrical and Computer Engineering. He received his PhD in Electrical Engineering and Computer Science from UC Berkeley and his BTech in Electrical Engineering from IIT Madras. His research interests lie in high dimensional statistics, optimization, signal processing, and information theory, and their applications to machine learning, reinforcement learning, and data science. Pananjady has received research awards from Adobe and Amazon, a Best Paper Prize (runner-up) for Young Researchers in Continuous Optimization from the Mathematical Optimization Society, the Lawrence D. Brown Award from the Institute of Mathematical Statistics, the David J. Sakrison Memorial Prize (for the best dissertation in EECS at Berkeley), and a Simons-Berkeley Research Fellowship in Probability, Geometry, and Computation in High Dimensions. His teaching has been recognized at both UC Berkeley and Georgia Tech.
Abstract:
Iterative algorithms are the workhorses of modern signal processing and statistical learning and are widely used to fit complex models to random data. While the choice of an algorithm and its hyperparameters determines both the speed and fidelity of the learning pipeline, it is common for this choice to be made heuristically, either by expensive trial-and-error or by comparing upper bounds on convergence rates of various candidate algorithms. Motivated by these issues, we develop a principled framework that produces sharp, iterate-by-iterate characterizations of solution quality for complex iterative algorithms on several nonconvex model-fitting problems with random data. Such sharp predictions can provide precise separations between families of algorithms while also revealing nonstandard convergence phenomena. We will showcase the general framework on several canonical models in statistical machine learning.
Ashwin Pananjady is an Assistant Professor at Georgia Tech, with a joint appointment between the Schools of Industrial and Systems Engineering and Electrical and Computer Engineering. He received his PhD in Electrical Engineering and Computer Science from UC Berkeley and his BTech in Electrical Engineering from IIT Madras. His research interests lie in high dimensional statistics, optimization, signal processing, and information theory, and their applications to machine learning, reinforcement learning, and data science. Pananjady has received research awards from Adobe and Amazon, a Best Paper Prize (runner-up) for Young Researchers in Continuous Optimization from the Mathematical Optimization Society, the Lawrence D. Brown Award from the Institute of Mathematical Statistics, the David J. Sakrison Memorial Prize (for the best dissertation in EECS at Berkeley), and a Simons-Berkeley Research Fellowship in Probability, Geometry, and Computation in High Dimensions. His teaching has been recognized at both UC Berkeley and Georgia Tech.
Abstract:
Iterative algorithms are the workhorses of modern signal processing and statistical learning and are widely used to fit complex models to random data. While the choice of an algorithm and its hyperparameters determines both the speed and fidelity of the learning pipeline, it is common for this choice to be made heuristically, either by expensive trial-and-error or by comparing upper bounds on convergence rates of various candidate algorithms. Motivated by these issues, we develop a principled framework that produces sharp, iterate-by-iterate characterizations of solution quality for complex iterative algorithms on several nonconvex model-fitting problems with random data. Such sharp predictions can provide precise separations between families of algorithms while also revealing nonstandard convergence phenomena. We will showcase the general framework on several canonical models in statistical machine learning.
Explore Similar Events
-
Loading Similar Events...