Presented By: Department of Statistics
Statistics Department Seminar Series: Colin Fogarty, Assistant Professor, Department of Statistics, University of Michigan
"Sensitivity Analysis in Observational Studies: Multiplicity and Heterogeneity"
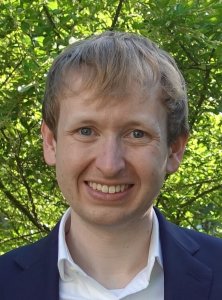
Abstract: A fundamental limitation of causal inference in observational studies is that perceived evidence for a treatment effect might instead be explained by factors not accounted for in the primary analysis. In a sensitivity analysis, the researcher determines how much hidden bias would need to exist to materially alter the study's conclusions. Once the results of a sensitivity analysis are reported, a critic seeking to undermine the observational study must argue that a proposed lurking variable impacts the odds of self-selection into treatment by at least the degree quantified by the sensitivity analysis, rather than simply proposing a variable for which adjustment did not occur.
When using covariate matching to adjust for overt bias, conventional approaches to sensitivity analysis consider inference for a single outcome variable under the presumption that the treatment effect is constant for all individuals. In the first part of the talk, we describe an approach to controlling the false discovery proportion in observational studies with multiple outcome variables while assessing robustness to unmeasured confounding: for any candidate subset of outcome variables, we provide sensitivity intervals for the proportion of outcomes within the set that are unaffected by the treatment. In the second portion of the talk, we introduce and compare recent proposals for extending methods for sensitivity analysis to heterogeneous treatment effects. In so doing, we highlight the role of conditional inverse probability weighting in improving the power of a sensitivity analysis.
https://sites.google.com/umich.edu/fogartyc
When using covariate matching to adjust for overt bias, conventional approaches to sensitivity analysis consider inference for a single outcome variable under the presumption that the treatment effect is constant for all individuals. In the first part of the talk, we describe an approach to controlling the false discovery proportion in observational studies with multiple outcome variables while assessing robustness to unmeasured confounding: for any candidate subset of outcome variables, we provide sensitivity intervals for the proportion of outcomes within the set that are unaffected by the treatment. In the second portion of the talk, we introduce and compare recent proposals for extending methods for sensitivity analysis to heterogeneous treatment effects. In so doing, we highlight the role of conditional inverse probability weighting in improving the power of a sensitivity analysis.
https://sites.google.com/umich.edu/fogartyc