Presented By: Aerospace Engineering
AE Defense: A framework to Augment Turbulence Models Using Full-field Inversion and Machine Learning
Aerospace Engineering PhD Candidate: Anand Pratap Singh, Dissertation Chair: Associate Professor Karthik Duraisamy
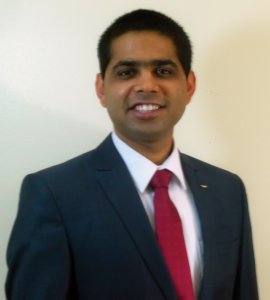
Aerospace Engineering PhD Candidate: Anand Pratap Singh, Dissertation Chair: Associate Professor Karthik Duraisamy
Abstract
Accurate prediction of turbulent flows remains a barrier to the widespread use of computational fluid dynamics for analysis and design. This thesis presents a generalized framework of physics-based data-augmented modeling, which we refer to as field-inversion and machine learning (FIML). FIML is utilized to develop augmentations to Reynolds-averaged Navier-Stokes based models using high fidelity simulation data and experimental measurements. This framework involves the solution of multiple inverse problems to infer spatial discrepancies in a baseline turbulence model. Several such discrepancy fields are used as inputs to a machine learning procedure, to reconstruct corrective functional forms in terms of local flow quantities. The machine-learned discrepancy is embedded in existing turbulence models and used in a predictive setting.
The FIML framework is applied to augment the Spalart-Allmaras (SA) and the Wilcox's k-omega model for flows involving curvature, adverse pressure gradients, and massive separation. The value of the framework is demonstrated in a number of applications, most notably by augmenting the SA model with the goal of improving separated flow predictions over airfoils using limited experimental data. The portability of the approach is demonstrated by embedding the data-augmented module developed using an in-house compressible finite-volume solver in a commercial incompressible finite-element solver. Overall, this work highlights the promise of using data to comprehensively augment physics-based models to obtain improved predictive models of complex phenomena.
Dissertation Committee:
Associate Professor Karthik Duraisamy, Chair
Professor Krishnakumar R. Garikipati, Cognate
Associate Professor Krzysztof J. Fidkowski
Professor Kenneth Powell
List of Publications
Journal
Machine Learning-augmented Predictive Modeling of Turbulent Separated Flows over Airfoils
AP Singh and S Medida and K Duraisamy, AIAA Journal, 2017
Using field inversion to quantify functional errors in turbulence closures
AP Singh and K Duraisamy, Physics of Fluids, 2016
Conference Proceedings
Data-driven augmentation of turbulence models for adverse pressure gradient flows
AP Singh and R Matai and K Duraisamy and P Durbin, AIAA Aviation, 2017
Characterizing and improving predictive accuracy in shock-turbulent boundary layer interactions using data-driven models
AP Singh and S Pan and K Duraisamy, AIAA SciTech, 2017
Augmentation of turbulence models using field inversion and machine learning
K Duraisamy and AP Singh and S Pan, AIAA SciTech, 2017
Informing Turbulence Closures With Computational and Experimental Data
K Duraisamy and AP Singh, AIAA SciTech, 2016
New approaches in turbulence and transition modeling using data-driven techniques
K Duraisamy and ZJ Zhang and AP Singh, AIAA SciTech, 2015
Multi Fidelity Aerodynamic Design of Ducted Microscale Rotor Systems
AP Singh and K Duraisamy, AHS Forum 70, 2014
Conference Presentations
Field Inversion and Machine learning for Predictive Turbulence Modeling
AP Singh and K Duraisamy, Advances in Turbulence Modeling Symposium, University of Michigan, 2017
Data-Driven Predictive Modeling of RANS Model Discrepancies. Invited.
AP Singh and K Duraisamy, SIAM Conference on Computational Science and Engineering, 2017
Machine Learning-Assisted Predictions of Turbulent Separated Flows over Airfoils
AP Singh and S Medida and K Duraisamy, 69th Annual APS DFD Meeting, 2016
Full field inversion: A tool to diagnose and improve closure models
AP Singh and K Duraisamy, 68th Annual APS DFD Meeting, 2015
Abstract
Accurate prediction of turbulent flows remains a barrier to the widespread use of computational fluid dynamics for analysis and design. This thesis presents a generalized framework of physics-based data-augmented modeling, which we refer to as field-inversion and machine learning (FIML). FIML is utilized to develop augmentations to Reynolds-averaged Navier-Stokes based models using high fidelity simulation data and experimental measurements. This framework involves the solution of multiple inverse problems to infer spatial discrepancies in a baseline turbulence model. Several such discrepancy fields are used as inputs to a machine learning procedure, to reconstruct corrective functional forms in terms of local flow quantities. The machine-learned discrepancy is embedded in existing turbulence models and used in a predictive setting.
The FIML framework is applied to augment the Spalart-Allmaras (SA) and the Wilcox's k-omega model for flows involving curvature, adverse pressure gradients, and massive separation. The value of the framework is demonstrated in a number of applications, most notably by augmenting the SA model with the goal of improving separated flow predictions over airfoils using limited experimental data. The portability of the approach is demonstrated by embedding the data-augmented module developed using an in-house compressible finite-volume solver in a commercial incompressible finite-element solver. Overall, this work highlights the promise of using data to comprehensively augment physics-based models to obtain improved predictive models of complex phenomena.
Dissertation Committee:
Associate Professor Karthik Duraisamy, Chair
Professor Krishnakumar R. Garikipati, Cognate
Associate Professor Krzysztof J. Fidkowski
Professor Kenneth Powell
List of Publications
Journal
Machine Learning-augmented Predictive Modeling of Turbulent Separated Flows over Airfoils
AP Singh and S Medida and K Duraisamy, AIAA Journal, 2017
Using field inversion to quantify functional errors in turbulence closures
AP Singh and K Duraisamy, Physics of Fluids, 2016
Conference Proceedings
Data-driven augmentation of turbulence models for adverse pressure gradient flows
AP Singh and R Matai and K Duraisamy and P Durbin, AIAA Aviation, 2017
Characterizing and improving predictive accuracy in shock-turbulent boundary layer interactions using data-driven models
AP Singh and S Pan and K Duraisamy, AIAA SciTech, 2017
Augmentation of turbulence models using field inversion and machine learning
K Duraisamy and AP Singh and S Pan, AIAA SciTech, 2017
Informing Turbulence Closures With Computational and Experimental Data
K Duraisamy and AP Singh, AIAA SciTech, 2016
New approaches in turbulence and transition modeling using data-driven techniques
K Duraisamy and ZJ Zhang and AP Singh, AIAA SciTech, 2015
Multi Fidelity Aerodynamic Design of Ducted Microscale Rotor Systems
AP Singh and K Duraisamy, AHS Forum 70, 2014
Conference Presentations
Field Inversion and Machine learning for Predictive Turbulence Modeling
AP Singh and K Duraisamy, Advances in Turbulence Modeling Symposium, University of Michigan, 2017
Data-Driven Predictive Modeling of RANS Model Discrepancies. Invited.
AP Singh and K Duraisamy, SIAM Conference on Computational Science and Engineering, 2017
Machine Learning-Assisted Predictions of Turbulent Separated Flows over Airfoils
AP Singh and S Medida and K Duraisamy, 69th Annual APS DFD Meeting, 2016
Full field inversion: A tool to diagnose and improve closure models
AP Singh and K Duraisamy, 68th Annual APS DFD Meeting, 2015
Explore Similar Events
-
Loading Similar Events...