Presented By: Department of Statistics
Statistics Department Seminar Series: Jason Xu, Department of Biomathematics, UCLA
“Enabling likelihood-based inference for complex and dependent data”
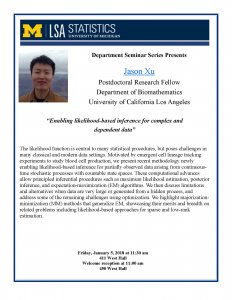
Abstract
The likelihood function is central to many statistical procedures, but poses challenges in many classical and modern data settings. Motivated by emergent cell lineage tracking experiments to study blood cell production, we present recent methodology newly enabling likelihood-based inference for partially observed data arising from continuous-time stochastic processes with countable state spaces. These computational advances allow principled inferential procedures such as maximum likelihood estimation, posterior inference, and expectation-maximization (EM) algorithms. We then discuss limitations and alternatives when data are very large or generated from a hidden process, and address some of the remaining challenges using optimization. We highlight majorization-minimization (MM) methods that generalize EM, showcasing their merits and breadth on related problems including likelihood-based approaches for sparse and low-rank estimation.
The likelihood function is central to many statistical procedures, but poses challenges in many classical and modern data settings. Motivated by emergent cell lineage tracking experiments to study blood cell production, we present recent methodology newly enabling likelihood-based inference for partially observed data arising from continuous-time stochastic processes with countable state spaces. These computational advances allow principled inferential procedures such as maximum likelihood estimation, posterior inference, and expectation-maximization (EM) algorithms. We then discuss limitations and alternatives when data are very large or generated from a hidden process, and address some of the remaining challenges using optimization. We highlight majorization-minimization (MM) methods that generalize EM, showcasing their merits and breadth on related problems including likelihood-based approaches for sparse and low-rank estimation.
Explore Similar Events
-
Loading Similar Events...