Presented By: Department of Statistics
Dissertation Defense: Outlier Identification in Spatio-Temporal Processes
Shrijita Bhattacharya
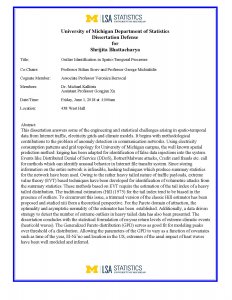
This dissertation answers some of the engineering and statistical challenges arising in spatio-temporal data from Internet traffic, electricity grids and climate models. It begins with methodological contributions to the problem of anomaly detection in communication networks. Using electricity consumption patterns and grid topology for University of Michigan campus, the well known spatial prediction method: kriging has been adapted for identification of false data injections into the system.
Events like Distributed Denial of Service (DDoS), Botnet/Malware attacks, Credit card frauds etc. call for methods which can identify unusual behavior in Internet file transfer system. Since storing information on the entire network is infeasible, hashing techniques which produce summary statistics for the network have been used. Owing to the rather heavy tailed nature of traffic payloads, extreme value theory (EVT) based techniques have been developed for identification of volumetric attacks from the summary statistics. These methods based on EVT require the estimation of the tail index of a heavy tailed distribution. The traditional estimators (Hill (1975) for the tail index tend to be biased in the presence of outliers. To circumvent this issue, a trimmed version of the classic Hill estimator has been proposed and studied xiii from a theoretical perspective. For the Pareto domain of attraction, the optimality and asymptotic normality of the estimator has been established. Additionally, a data driven strategy to detect the number of extreme outliers in heavy tailed data has also been presented. The dissertation concludes with the statistical formulation of m-year return levels of extreme climatic events (heat/cold waves). The Generalized Pareto distribution (GPD) serves as good fit for modeling peaks over threshold of a distribution. Allowing the parameters of the GPD to vary as a function of covariates such as time of the year, El-Ni˜no and location in the US, extremes of the areal impact of heat waves have been well modeled and inferred.
Events like Distributed Denial of Service (DDoS), Botnet/Malware attacks, Credit card frauds etc. call for methods which can identify unusual behavior in Internet file transfer system. Since storing information on the entire network is infeasible, hashing techniques which produce summary statistics for the network have been used. Owing to the rather heavy tailed nature of traffic payloads, extreme value theory (EVT) based techniques have been developed for identification of volumetric attacks from the summary statistics. These methods based on EVT require the estimation of the tail index of a heavy tailed distribution. The traditional estimators (Hill (1975) for the tail index tend to be biased in the presence of outliers. To circumvent this issue, a trimmed version of the classic Hill estimator has been proposed and studied xiii from a theoretical perspective. For the Pareto domain of attraction, the optimality and asymptotic normality of the estimator has been established. Additionally, a data driven strategy to detect the number of extreme outliers in heavy tailed data has also been presented. The dissertation concludes with the statistical formulation of m-year return levels of extreme climatic events (heat/cold waves). The Generalized Pareto distribution (GPD) serves as good fit for modeling peaks over threshold of a distribution. Allowing the parameters of the GPD to vary as a function of covariates such as time of the year, El-Ni˜no and location in the US, extremes of the areal impact of heat waves have been well modeled and inferred.
Explore Similar Events
-
Loading Similar Events...