Presented By: Department of Statistics
Dissertation Defense: On High-Dimensional Misspecified Quantile Regression
Alexander Giessing
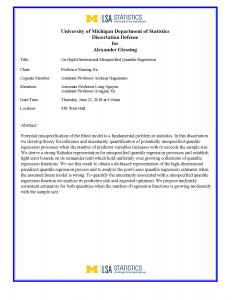
Potential misspecification of the fitted model is a fundamental problem in statistics. In this dissertation we develop theory for inference and uncertainty quantification of potentially misspecified quantile regression processes when the number of predictor variables increases with or exceeds the sample size. We derive a strong Bahadur representation for misspecified quantile regression processes and establish tight error bounds on its remainder term which hold uniformly over growing collections of quantile regression functions. We use this result to obtain a de-biased representation of the high-dimensional penalized quantile regression process and to analyze the post-Lasso quantile regression estimator when the assumed linear model is wrong. To quantify the uncertainty associated with a misspecified quantile regression function we analyze its predictive risk and expected optimism. We propose uniformly consistent estimators for both quantities when the number of regression functions is growing moderately with the sample size.
Explore Similar Events
-
Loading Similar Events...