Presented By: Department of Statistics
Statistics Department Seminar Series: Jessi Cisewski, Assistant Professor of Statistics & Data Science, Yale University
Investigating Spatially Complex Data with Topological Data Analysis
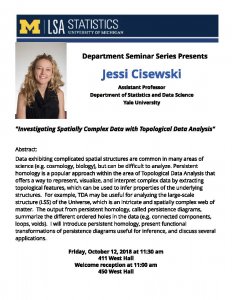
Data exhibiting complicated spatial structures are common in many areas of science (e.g. cosmology, biology), but can be difficult to analyze. Persistent homology is a popular approach within the area of Topological Data Analysis that offers a way to represent, visualize, and interpret complex data by extracting topological features, which can be used to infer properties of the underlying structures. For example, TDA may be useful for analyzing the large-scale structure (LSS) of the Universe, which is an intricate and spatially complex web of matter. The output from persistent homology, called persistence diagrams, summarize the different ordered holes in the data (e.g. connected components, loops, voids). I will introduce persistent homology, present functional transformations of persistence diagrams useful for inference, and discuss several applications.
Explore Similar Events
-
Loading Similar Events...