Presented By: Department of Psychology
Cognition & Cognitive Neuroscience Forum
Matt Jones, Associate Professor of Psychology & Neuroscience, University of Colorado (Boulder)
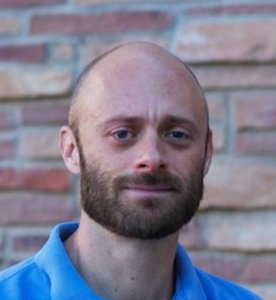
Diffusion models have shown great success in explaining choice response time in a wide variety of domains. However, to account for RT differences between correct and error responses, the model must assume random variability in parameters across trials. The first part of this talk presents mathematical results showing that, if this variability is unconstrained, then the model becomes unfalsifiable.
The second part presents a positive theory of intertrial variability that resolves this problem of excess flexibility, based on an integration of the diffusion model with reinforcement learning. These are arguably the two most successful frameworks in cognitive modeling, respectively describing within-trial and across-trial dynamics. I provide a Bayesian derivation that yields a natural synthesis of the two models and makes novel predictions about bidirectional influences between learning and decision making. Fits to data show the model simultaneously accounts for choice and RT within trials, learning across trials, and various interdependencies between these two timescales.
The second part presents a positive theory of intertrial variability that resolves this problem of excess flexibility, based on an integration of the diffusion model with reinforcement learning. These are arguably the two most successful frameworks in cognitive modeling, respectively describing within-trial and across-trial dynamics. I provide a Bayesian derivation that yields a natural synthesis of the two models and makes novel predictions about bidirectional influences between learning and decision making. Fits to data show the model simultaneously accounts for choice and RT within trials, learning across trials, and various interdependencies between these two timescales.
Explore Similar Events
-
Loading Similar Events...