Presented By: Department of Statistics
Statistics Department Seminar Series: Qingyuan Zhao, Department of Statistics, Wharton School, University of Pennsylvania
Mendelian randomization: A comprehensive statistical approach and applications to preventing heart disease
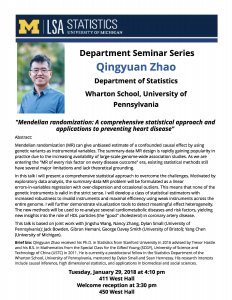
Mendelian randomization (MR) can give unbiased estimate of a confounded causal effect by using genetic variants as instrumental variables. The summary-data MR design is rapidly gaining popularity in practice due to the increasing availability of large-scale genome-wide association studies. As we are entering the "MR of every risk factor on every disease outcome" era, existing statistical methods still have several major limitations and lack theoretical grounding.
In this talk I will present a comprehensive statistical approach to overcome the challenges. Motivated by exploratory data analysis, the summary-data MR problem will be formulated as a linear errors-in-variables regression with over-dispersion and occasional outliers. This means that none of the genetic instruments is valid in the strict sense. I will develop a class of statistical estimators with increased robustness to invalid instruments and maximal efficiency using weak instruments across the entire genome. I will further demonstrate visualization tools to detect meaningful effect heterogeneity. The new methods will be used to re-analyze several cardiometabolic diseases and risk factors, yielding new insights into the role of HDL particles (the "good" cholesterol) in coronary artery disease.
This talk is based on joint work with Jingshu Wang, Nancy Zhang, Dylan Small (University of Pennsylvania); Jack Bowden, Gibran Hemani, George Davey Smith (University of Bristol); Yang Chen (University of Michigan).
Brief bio: Qingyuan Zhao received his Ph.D. in Statistics from Stanford University in 2016 advised by Trevor Hastie and his B.S. in Mathematics from the Special Class for the Gifted Young (SCGY), University of Science and Technology of China (USTC) in 2011. He is currently a postdoctoral fellow in the Statistics Department of the Wharton School, University of Pennsylvania, mentored by Dylan Small and Sean Hennessy. His research interests include causal inference, high dimensional statistics, and applications in biomedical and social sciences.
In this talk I will present a comprehensive statistical approach to overcome the challenges. Motivated by exploratory data analysis, the summary-data MR problem will be formulated as a linear errors-in-variables regression with over-dispersion and occasional outliers. This means that none of the genetic instruments is valid in the strict sense. I will develop a class of statistical estimators with increased robustness to invalid instruments and maximal efficiency using weak instruments across the entire genome. I will further demonstrate visualization tools to detect meaningful effect heterogeneity. The new methods will be used to re-analyze several cardiometabolic diseases and risk factors, yielding new insights into the role of HDL particles (the "good" cholesterol) in coronary artery disease.
This talk is based on joint work with Jingshu Wang, Nancy Zhang, Dylan Small (University of Pennsylvania); Jack Bowden, Gibran Hemani, George Davey Smith (University of Bristol); Yang Chen (University of Michigan).
Brief bio: Qingyuan Zhao received his Ph.D. in Statistics from Stanford University in 2016 advised by Trevor Hastie and his B.S. in Mathematics from the Special Class for the Gifted Young (SCGY), University of Science and Technology of China (USTC) in 2011. He is currently a postdoctoral fellow in the Statistics Department of the Wharton School, University of Pennsylvania, mentored by Dylan Small and Sean Hennessy. His research interests include causal inference, high dimensional statistics, and applications in biomedical and social sciences.
Explore Similar Events
-
Loading Similar Events...