Presented By: U-M Industrial & Operations Engineering
IOE Lunch & Learn Seminar Series: Xubu Yue and Seokhyun Chung
Two presentations related to Multivariate Gaussian Processes and Functional Principal Component Analysis
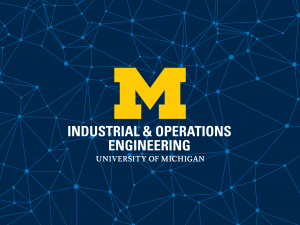
Open to all IOE graduate students and faculty. Lunch will be provided. In order to get an accurate count for food, please RSVP by noon on Wednesday, February 20.
1st Presentation: Xubo Yue on "Variational Inference of Joint Models using Multivariate Gaussian Convolution Processes"
Abstract:
In recent years, the multivariate Gaussian process (MGP) has drawn significant attention as an efficient non-parametric approach to predict longitudinal signal trajectories. We would like to exploit the MGP to explore the following question: can we use both time-to-event data (also known as survival data) along with predicted longitudinal signals to obtain a reliable event prediction? In this work, we present a non-parametric prognostic framework for individualized event prediction based on joint modeling of both longitudinal and time-to-event data. Our approach exploits a multivariate Gaussian convolution process (MGCP) to model the evolution of longitudinal signals and a Cox model to map time-to-event data with longitudinal data modeled through MGCP. Taking advantage of the unique structure imposed by convolved processes, we provide a variational inference framework to simultaneously estimate parameters in the joint MGCP-Cox model. This facilitates scalability to large data settings and safeguards against model overfitting. Experiments on synthetic and real-world data show that the proposed framework outperforms state-of-the-art approaches built on two-stage inference and strong parametric assumptions.
Bio:
Xubo Yue is a first-year Ph.D. student in the Industrial and Operations Engineering Department at the University of Michigan, Ann Arbor. He holds a Master’s degree in Biostatistics from the University of Michigan, and Bachelor’s degree in Biomedical Sciences and Applied Mathematics from the University of Macau. Xubo’s current research focuses on Multivariate Gaussian Processes and Bayesian optimization for reinforcement learning.
2nd Presentation: Seokhyun Chung on "Functional Principal Component Analysis for Extrapolating Multi-stream Longitudinal Data"
Abstract:
The advance of modern sensor technologies enables collection of multi-stream longitudinal data where multiple signals from different units are collected in real-time. In this article, we present a non-parametric approach to predict the evolution of multi-stream longitudinal data for an in-service unit through borrowing strength from other historical units. Our approach first decomposes each stream into a linear combination of eigenfunctions and their corresponding functional principal component (FPC) scores. A Gaussian process prior for the FPC scores is then established based on a functional semi-metric that measures similarities between streams of historical units and the in-service unit. Finally, an empirical Bayesian updating strategy is derived to update the established prior using real-time stream data obtained from the in-service unit. Experiments on synthetic and real-world data show that the proposed framework outperforms state-of-the-art approaches and can effectively account for heterogeneity as well as achieve high predictive accuracy.
Bio:
Seokhyun Chung is a first-year Ph.D. student in the Industrial and Operations Engineering Department at the University of Michigan, Ann Arbor. He holds Master’s and Bachelor’s degree in industrial and management engineering from the Korea University. Seokhyun’s current research interests include development of machine learning and Bayesian non-parametric models in order to analyze large-scale data collected from IoT devices in connected environments such as smart factories, wearable devices, and battery management system for electric vehicles.
1st Presentation: Xubo Yue on "Variational Inference of Joint Models using Multivariate Gaussian Convolution Processes"
Abstract:
In recent years, the multivariate Gaussian process (MGP) has drawn significant attention as an efficient non-parametric approach to predict longitudinal signal trajectories. We would like to exploit the MGP to explore the following question: can we use both time-to-event data (also known as survival data) along with predicted longitudinal signals to obtain a reliable event prediction? In this work, we present a non-parametric prognostic framework for individualized event prediction based on joint modeling of both longitudinal and time-to-event data. Our approach exploits a multivariate Gaussian convolution process (MGCP) to model the evolution of longitudinal signals and a Cox model to map time-to-event data with longitudinal data modeled through MGCP. Taking advantage of the unique structure imposed by convolved processes, we provide a variational inference framework to simultaneously estimate parameters in the joint MGCP-Cox model. This facilitates scalability to large data settings and safeguards against model overfitting. Experiments on synthetic and real-world data show that the proposed framework outperforms state-of-the-art approaches built on two-stage inference and strong parametric assumptions.
Bio:
Xubo Yue is a first-year Ph.D. student in the Industrial and Operations Engineering Department at the University of Michigan, Ann Arbor. He holds a Master’s degree in Biostatistics from the University of Michigan, and Bachelor’s degree in Biomedical Sciences and Applied Mathematics from the University of Macau. Xubo’s current research focuses on Multivariate Gaussian Processes and Bayesian optimization for reinforcement learning.
2nd Presentation: Seokhyun Chung on "Functional Principal Component Analysis for Extrapolating Multi-stream Longitudinal Data"
Abstract:
The advance of modern sensor technologies enables collection of multi-stream longitudinal data where multiple signals from different units are collected in real-time. In this article, we present a non-parametric approach to predict the evolution of multi-stream longitudinal data for an in-service unit through borrowing strength from other historical units. Our approach first decomposes each stream into a linear combination of eigenfunctions and their corresponding functional principal component (FPC) scores. A Gaussian process prior for the FPC scores is then established based on a functional semi-metric that measures similarities between streams of historical units and the in-service unit. Finally, an empirical Bayesian updating strategy is derived to update the established prior using real-time stream data obtained from the in-service unit. Experiments on synthetic and real-world data show that the proposed framework outperforms state-of-the-art approaches and can effectively account for heterogeneity as well as achieve high predictive accuracy.
Bio:
Seokhyun Chung is a first-year Ph.D. student in the Industrial and Operations Engineering Department at the University of Michigan, Ann Arbor. He holds Master’s and Bachelor’s degree in industrial and management engineering from the Korea University. Seokhyun’s current research interests include development of machine learning and Bayesian non-parametric models in order to analyze large-scale data collected from IoT devices in connected environments such as smart factories, wearable devices, and battery management system for electric vehicles.
Explore Similar Events
-
Loading Similar Events...