Presented By: Department of Statistics
Dissertation Defense: Approaches for identifying biases in single-cell RNA-sequencing data
Julie Deeke
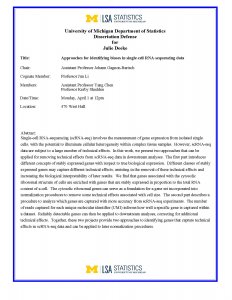
Single-cell RNA-sequencing (scRNA-seq) involves the measurement of gene expression from isolated single cells, with the potential to illuminate cellular heterogeneity within complex tissue samples. However, scRNA-seq data are subject to a large number of technical effects. In this work, we present two approaches that can be applied for removing technical effects from scRNA-seq data in downstream analyses. The first part introduces different concepts of stably expressed genes with respect to true biological expression. Different classes of stably expressed genes may capture different technical effects, assisting in the removal of these technical effects and increasing the biological interpretability of later results. We find that genes associated with the cytosolic ribosomal structure of cells are enriched with genes that are stably expressed in proportion to the total RNA content of a cell. The cytosolic ribosomal genes can serve as a foundation for a gene set incorporated into normalization procedures to remove some technical effects associated with cell size. The second part describes a procedure to analyze which genes are captured with more accuracy from scRNA-seq experiments. The number of reads captured for each unique molecular identifier (UMI) informs how well a specific gene is captured within a dataset. Reliably detectable genes can then be applied to downstream analyses, correcting for additional technical effects. Together, these two projects provide two approaches to identifying genes that capture technical effects in scRNA-seq data and can be applied to later normalization procedures.