Presented By: Computer Science and Engineering Division
CSE Distinguished Lecture Series--Physics, Machine Learning, and Networks
Cris Moore, Santa Fe Institute
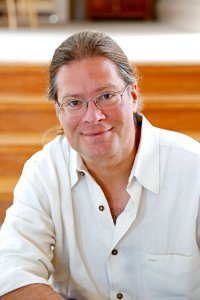
There is a deep analogy between Bayesian inference — where we try to fit a model to data, which has a ground-truth structure partly hidden by noise — and statistical physics. Many concepts like energy landscapes, free energy, and phase transitions can be usefully carried over from physics to machine learning and computer science. At the very least, these techniques are a source of conjectures that have stimulated new work in probability, combinatorics, and theoretical computer science. At their best, they offer strong intuitions about the structure of inference problems and possible algorithms for them.
One recent success of this interface is the discovery of a phase transition in community detection in sparse graphs. Analogous transitions exist in many other inference problems, where our ability to find patterns in data jumps suddenly as a function of how noisy they are. I will discuss why and how this detectability transition occurs, review what is known rigorously, and present a number of open questions that cry out for proofs.
One recent success of this interface is the discovery of a phase transition in community detection in sparse graphs. Analogous transitions exist in many other inference problems, where our ability to find patterns in data jumps suddenly as a function of how noisy they are. I will discuss why and how this detectability transition occurs, review what is known rigorously, and present a number of open questions that cry out for proofs.
Explore Similar Events
-
Loading Similar Events...