Presented By: Nuclear Engineering & Radiological Sciences
PhD Defense: Haining Zhou
Sparse Functional Expansion Based Method for Solving High-dimensional Uncertainty Quantification Problems and Its Application to the Nuclear Transient Test Reactor (TREAT)
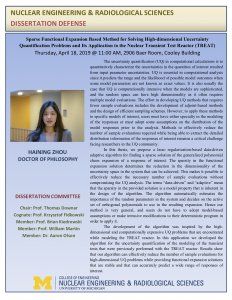
Title: Sparse Functional Expansion Based Method for Solving High-dimensional Uncertainty Quantification Problems and Its Application to the Nuclear Transient Test Reactor (TREAT)
Chair: Prof. Thomas Downar
Abstract: The uncertainty quantification (UQ) in computational calculations is to quantitatively characterize the uncertainties in the quantities of interest resulted from input parameter uncertainties. UQ is essential in computational analysis since it predicts the range and the likelihood of possible model outcomes when some model parameters are not known as exact values. It is also usually the case that UQ is computationally intensive when the models are sophisticated, and the random space can have high dimensionality as it often requires multiple model evaluations. The effort in developing UQ methods that requires fewer sample evaluations includes the development of adjoint-based methods and the design of efficient sampling schemes. However, to apply these methods to specific models of interest, users must have either specialty in the modeling of the responses or must adopt some assumptions on the distribution of the model responses prior to the analysis. Methods to effectively reduce the number of sample evaluations required while being able to extract the detailed distribution information of the responses of interest remains a critical challenge facing researchers in the UQ community.
In this thesis, we propose a lasso regularization-based data-driven adaptive algorithm for finding a sparse solution of the generalized polynomial chaos expansion of a response of interest. The sparsity in the functional expansion solution determines the reduction in the dimensionality of the uncertainty space in the system that can be achieved. This makes it possible to effectively reduce the necessary number of sample evaluations without compromising the UQ analysis. The terms “data-driven” and “adaptive” mean that the sparsity in the provided solution is a model property that is inherent in the design of the algorithm. The algorithm automatically estimates the importance of the random parameters in the system and decides on the active set of orthogonal polynomials to use in the resulting expansion. Hence our method is very general, and users do not have to adopt model-based assumptions or make intrusive modifications to their deterministic program in order to apply it.
The development of the algorithm was inspired by the high-dimensional and computationally expensive UQ problems that are encountered while modeling the TREAT reactor. In this application we developed the algorithm for the uncertainty quantification of the modeling of the transient tests that were previously performed with the TREAT reactor. Results show that our algorithm can effectively reduce the number of sample evaluations for high-dimensional UQ problems while providing functional expansion solutions that are stable and that can accurately predict a wide range of responses of interest.
Chair: Prof. Thomas Downar
Abstract: The uncertainty quantification (UQ) in computational calculations is to quantitatively characterize the uncertainties in the quantities of interest resulted from input parameter uncertainties. UQ is essential in computational analysis since it predicts the range and the likelihood of possible model outcomes when some model parameters are not known as exact values. It is also usually the case that UQ is computationally intensive when the models are sophisticated, and the random space can have high dimensionality as it often requires multiple model evaluations. The effort in developing UQ methods that requires fewer sample evaluations includes the development of adjoint-based methods and the design of efficient sampling schemes. However, to apply these methods to specific models of interest, users must have either specialty in the modeling of the responses or must adopt some assumptions on the distribution of the model responses prior to the analysis. Methods to effectively reduce the number of sample evaluations required while being able to extract the detailed distribution information of the responses of interest remains a critical challenge facing researchers in the UQ community.
In this thesis, we propose a lasso regularization-based data-driven adaptive algorithm for finding a sparse solution of the generalized polynomial chaos expansion of a response of interest. The sparsity in the functional expansion solution determines the reduction in the dimensionality of the uncertainty space in the system that can be achieved. This makes it possible to effectively reduce the necessary number of sample evaluations without compromising the UQ analysis. The terms “data-driven” and “adaptive” mean that the sparsity in the provided solution is a model property that is inherent in the design of the algorithm. The algorithm automatically estimates the importance of the random parameters in the system and decides on the active set of orthogonal polynomials to use in the resulting expansion. Hence our method is very general, and users do not have to adopt model-based assumptions or make intrusive modifications to their deterministic program in order to apply it.
The development of the algorithm was inspired by the high-dimensional and computationally expensive UQ problems that are encountered while modeling the TREAT reactor. In this application we developed the algorithm for the uncertainty quantification of the modeling of the transient tests that were previously performed with the TREAT reactor. Results show that our algorithm can effectively reduce the number of sample evaluations for high-dimensional UQ problems while providing functional expansion solutions that are stable and that can accurately predict a wide range of responses of interest.