Presented By: Department of Statistics Dissertation Defenses
Dissertation Defense: Statistical Learning for Networks with Node Features
Boang Liu
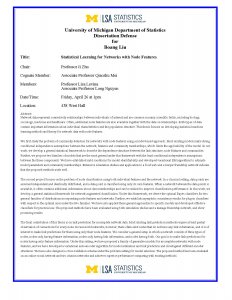
Network data represent connectivity relationships between individuals of interest and are common in many scientific fields, including biology, sociology, medicine and healthcare. Often, additional node features are also available together with the data on relationships. Both types of data contain important information about individual characteristics and the population structure. This thesis focuses on developing statistical machine learning methods and theory for network data with node features.
We first study the problem of community detection for networks with node features using a model-based approach. Most existing models make strong conditional independence assumptions between the network, features and community memberships, which limits the applicability of the model. In our work, we develop a general statistical framework to describe the dependence structure between the link structure, node features and communities. Further, we propose two families of models that are the most general under this framework with the least conditional independence assumptions between the three components. We have established mild conditions for model identifiability and developed variational EM algorithms to estimate model parameters and community memberships. Extensive simulation studies and application to a food web and a lawyer friendship network indicate that the proposed methods work well.
The second project focuses on the problem of node classification using both individual features and the network. In a classical setting, data points are assumed independent and identically distributed, and a data point is classified using only its own features. When a network between the data points is available, it often contains additional information about class memberships and can be utilized to improve classification performance. In this work, we develop a general statistical framework for network augmented classification. Under this framework, we derive the optimal Bayes classifiers for two general families of distributions incorporating node features and networks. Further, we establish asymptotic consistency results for plug-in classifiers with respect to the optimal ones under the two families. We have also applied these general approaches to specific models and developed effective classifiers for practical use. The proposed methods have been evaluated using both simulation studies and a teenage friendship network, and show promising results.
The final contribution of this thesis is on link prediction for incomplete network data. Most existing link prediction methods require at least partial observation of connections for every node. In real-world networks, however, there often exist nodes that do not have any link information, and it is of interest to make link predictions for them using only their node features. We consider a general setup in which a network consists of three types of nodes, nodes only having feature information, nodes only having link information, and nodes having both. Our goal is to make link predictions for nodes having only feature information. Under this setting, we have proposed a family of generative models for incomplete networks with node features, and we have developed a variational auto-encoder algorithm for model estimation and link prediction and investigated different encoder structures. We have also designed a cross-validation scheme under the problem setting for model selection. The proposed method has been evaluated on an online social network and two citation networks and achieves superior performance comparing with existing methods.
We first study the problem of community detection for networks with node features using a model-based approach. Most existing models make strong conditional independence assumptions between the network, features and community memberships, which limits the applicability of the model. In our work, we develop a general statistical framework to describe the dependence structure between the link structure, node features and communities. Further, we propose two families of models that are the most general under this framework with the least conditional independence assumptions between the three components. We have established mild conditions for model identifiability and developed variational EM algorithms to estimate model parameters and community memberships. Extensive simulation studies and application to a food web and a lawyer friendship network indicate that the proposed methods work well.
The second project focuses on the problem of node classification using both individual features and the network. In a classical setting, data points are assumed independent and identically distributed, and a data point is classified using only its own features. When a network between the data points is available, it often contains additional information about class memberships and can be utilized to improve classification performance. In this work, we develop a general statistical framework for network augmented classification. Under this framework, we derive the optimal Bayes classifiers for two general families of distributions incorporating node features and networks. Further, we establish asymptotic consistency results for plug-in classifiers with respect to the optimal ones under the two families. We have also applied these general approaches to specific models and developed effective classifiers for practical use. The proposed methods have been evaluated using both simulation studies and a teenage friendship network, and show promising results.
The final contribution of this thesis is on link prediction for incomplete network data. Most existing link prediction methods require at least partial observation of connections for every node. In real-world networks, however, there often exist nodes that do not have any link information, and it is of interest to make link predictions for them using only their node features. We consider a general setup in which a network consists of three types of nodes, nodes only having feature information, nodes only having link information, and nodes having both. Our goal is to make link predictions for nodes having only feature information. Under this setting, we have proposed a family of generative models for incomplete networks with node features, and we have developed a variational auto-encoder algorithm for model estimation and link prediction and investigated different encoder structures. We have also designed a cross-validation scheme under the problem setting for model selection. The proposed method has been evaluated on an online social network and two citation networks and achieves superior performance comparing with existing methods.
Explore Similar Events
-
Loading Similar Events...