Presented By: Department of Statistics Dissertation Defenses
Dissertation Defense: Scalable classification methods with applications to healthcare claims and automotive dealership data
Wenyi Wu
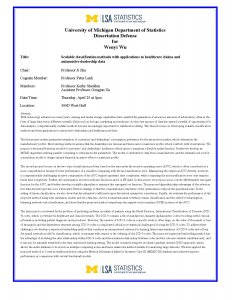
With technology advances in recent years, sensing and media storage capabilities have enabled the generation of enormous amounts of information, often in the form of large data sets in different scientific fields such as biology, marketing and medicine. As this vast amount of data has opened a wealth of opportunities for data analysis, computationally scalable methods become increasingly important for statistical modeling. This thesis focuses on developing scalable classification methods and their applications to automotive dealerships and healthcare problems.
The first project studies parameter estimation of customers' and dealerships' consumption preference for the automotive market, which determines the manufacturers' profits. Most existing methods assume that the dealerships are rational and hence aim to maximize profits, which conflicts with observations. We propose a structural Bayesian model for customers’ and dealerships’ preference which aims to maximize a flexible utility function. Further we develop an MCMC algorithm utilizing parallel computing to estimate model parameters. The model is calibrated to data from a manufacturer, and the estimates are used in a simulation model to design optimal financial incentive offers to maximize profits.
The second project focuses on the two-class classification problem based on the area under the receiver operating curve (AUC), which is often considered as a more comprehensive measure for the performance of a classifier comparing with the misclassification error. Maximizing the empirical AUC directly, however, is computationally challenging as naive computation of the AUC requires quadratic time complexity, while computing the misclassification error only requires linear time complexity. Further, the optimization involves indicator functions and it is NP-hard. In this project, we propose a non-convex differentiable surrogate function for the AUC, and further develop a scalable algorithm to optimize this surrogate loss function. The proposed algorithm takes advantage of the selection tree data structure and also uses a truncated Newton strategy so that the computational complexity of the optimization scales at the quasilinear time. In the setting of linear classification, we also show that the estimated coefficients enjoy theoretical asymptotic consistency. Finally, we evaluate the performance of the proposed method using both simulation studies and two data sets, one for normal/abnormal vertebral column classification and the other for behaving/not-behaving network visit classification, and show that the proposed method outperforms the support vector machine (SVM) in terms of the AUC.
The last project is motivated by the problem of predicting midterm mortality of patients using the Ninth Revision, International Classification of Diseases (ICD-9) codes, which is relevant for healthcare and clinical research. The ICD-9 contains a list of standard six-character alphanumeric codes recording useful clinical information including patient diagnoses and procedures. However, the number of ICD-9 codes in a specific study is often large, on the order of thousands or tens of thousands, and the dependence structure among ICD-9 codes is complicated, which pose statistical challenges for using the ICD-9 codes. To address these challenges, we develop a supervised embedding method that combines an unsupervised criterion for learning latent representations of ICD-9 codes and a Deep Set neural network model for classification, which is invariant with respect to the ordering of the ICD-9 codes. The proposed supervised embedding method has the advantage of modeling the inter-relationship within ICD-9 codes and the nonlinear relationship between codes and the outcome variable simultaneously, and it can also be naturally extended to the semi-supervised learning setting. The model is trained using the stochastic gradient descent (SGD) approach, which allows the entire database to be stored on multiple computing nodes and hence makes the method suitable for analyzing large data sets. We have applied the proposed method to 1-year mortality prediction using the Medical Information Mart for Incentive Care III (MIMIC-III) database and achieved superior performance in comparison with several benchmark models.
The first project studies parameter estimation of customers' and dealerships' consumption preference for the automotive market, which determines the manufacturers' profits. Most existing methods assume that the dealerships are rational and hence aim to maximize profits, which conflicts with observations. We propose a structural Bayesian model for customers’ and dealerships’ preference which aims to maximize a flexible utility function. Further we develop an MCMC algorithm utilizing parallel computing to estimate model parameters. The model is calibrated to data from a manufacturer, and the estimates are used in a simulation model to design optimal financial incentive offers to maximize profits.
The second project focuses on the two-class classification problem based on the area under the receiver operating curve (AUC), which is often considered as a more comprehensive measure for the performance of a classifier comparing with the misclassification error. Maximizing the empirical AUC directly, however, is computationally challenging as naive computation of the AUC requires quadratic time complexity, while computing the misclassification error only requires linear time complexity. Further, the optimization involves indicator functions and it is NP-hard. In this project, we propose a non-convex differentiable surrogate function for the AUC, and further develop a scalable algorithm to optimize this surrogate loss function. The proposed algorithm takes advantage of the selection tree data structure and also uses a truncated Newton strategy so that the computational complexity of the optimization scales at the quasilinear time. In the setting of linear classification, we also show that the estimated coefficients enjoy theoretical asymptotic consistency. Finally, we evaluate the performance of the proposed method using both simulation studies and two data sets, one for normal/abnormal vertebral column classification and the other for behaving/not-behaving network visit classification, and show that the proposed method outperforms the support vector machine (SVM) in terms of the AUC.
The last project is motivated by the problem of predicting midterm mortality of patients using the Ninth Revision, International Classification of Diseases (ICD-9) codes, which is relevant for healthcare and clinical research. The ICD-9 contains a list of standard six-character alphanumeric codes recording useful clinical information including patient diagnoses and procedures. However, the number of ICD-9 codes in a specific study is often large, on the order of thousands or tens of thousands, and the dependence structure among ICD-9 codes is complicated, which pose statistical challenges for using the ICD-9 codes. To address these challenges, we develop a supervised embedding method that combines an unsupervised criterion for learning latent representations of ICD-9 codes and a Deep Set neural network model for classification, which is invariant with respect to the ordering of the ICD-9 codes. The proposed supervised embedding method has the advantage of modeling the inter-relationship within ICD-9 codes and the nonlinear relationship between codes and the outcome variable simultaneously, and it can also be naturally extended to the semi-supervised learning setting. The model is trained using the stochastic gradient descent (SGD) approach, which allows the entire database to be stored on multiple computing nodes and hence makes the method suitable for analyzing large data sets. We have applied the proposed method to 1-year mortality prediction using the Medical Information Mart for Incentive Care III (MIMIC-III) database and achieved superior performance in comparison with several benchmark models.