Presented By: Department of Statistics Dissertation Defenses
Dissertation Defense: Just-In-Time Adaptive Interventions: Experiment, Inference and Online Learning
Peng Liao
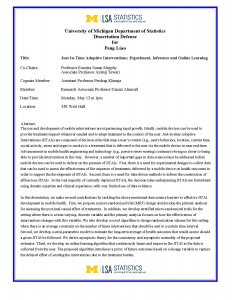
The use and development of mobile interventions are experiencing rapid growth. Ideally, mobile devices can be used to provide treatment/support whenever needed and to adapt treatment to the context of the user. Just-in-time Adaptive Interventions (JITAIs) are composed of decision rules that map a user’s context (e.g., user's behaviors, location, current time, social activity, stress and urges to smoke) to a treatment that is delivered to the user via the mobile device in near real-time. Advancements in mobile health engineering and technology (e.g., passive stress sensing) continue to bring us closer to being able to provide interventions in this way. However, a number of important gaps in data science must be addressed before mobile devices can be used to deliver on the promise of JITAIs. First, there is a need for experimental designs to collect data that can be used to assess the effectiveness of the sequence of treatments delivered by a mobile device on health outcomes in order to support the development of JITAIs. Second, there is a need for data-driven methods to inform the construction of efficacious JITAIs. In the vast majority of currently deployed JITAIs, the decision rules underpinning JITAIs are formulated using domain expertise and clinical experience, with very limited use of data evidence.
In this dissertation, we make several contributions by tackling the above mentioned data science barriers to effective JITAI development in mobile health. First, we propose a micro-randomized trial (MRT) design and develop the primary analysis for assessing the proximal causal effect of treatments. In addition, we develop stratified micro-randomized trials for the setting where there is a time-varying, discrete variable and the primary analysis focuses on how the effectiveness of interventions changes with this variable. We also develop a novel algorithm to design randomization scheme for this setting when there is an average constraint on the number of times interventions that should be sent in a certain time interval. Second, we develop a semi-parametric model to estimate the long-term average of health outcomes that would accrue should a given JITAI be followed. We derive asymptotic theory for the consistency and asymptotic normality of the proposed estimator. Third, we develop an online learning algorithm that continuously learns and improves the JITAI as the data is collected from the user. The proposed algorithm introduces a proxy of future outcomes based on a dosage variable to capture the delayed effect of sending the interventions due to the treatment burden.
In this dissertation, we make several contributions by tackling the above mentioned data science barriers to effective JITAI development in mobile health. First, we propose a micro-randomized trial (MRT) design and develop the primary analysis for assessing the proximal causal effect of treatments. In addition, we develop stratified micro-randomized trials for the setting where there is a time-varying, discrete variable and the primary analysis focuses on how the effectiveness of interventions changes with this variable. We also develop a novel algorithm to design randomization scheme for this setting when there is an average constraint on the number of times interventions that should be sent in a certain time interval. Second, we develop a semi-parametric model to estimate the long-term average of health outcomes that would accrue should a given JITAI be followed. We derive asymptotic theory for the consistency and asymptotic normality of the proposed estimator. Third, we develop an online learning algorithm that continuously learns and improves the JITAI as the data is collected from the user. The proposed algorithm introduces a proxy of future outcomes based on a dosage variable to capture the delayed effect of sending the interventions due to the treatment burden.
Explore Similar Events
-
Loading Similar Events...