Presented By: Department of Statistics
Statistics Department Seminar Series: Kengo Kato, Associate Professor, Department of Statistics and Data Science, Cornell University
"High dimensional CLT and bootstrap for incomplete U-statistics with extension"
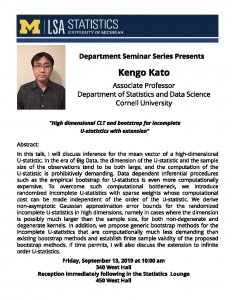
In this talk, I will discuss inference for the mean vector of a high
dimensional U-statistic. In the era of Big Data, the dimension of the U statistic and the sample size of the observations tend to be both large, and the computation of the U-statistic is prohibitively demanding. Data-dependent inferential procedures such as the empirical bootstrap for U-statistics is even more computationally expensive. To overcome such computational bottleneck, we introduce randomized incomplete U-statistics with sparse weights whose computational cost can be made independent of the order of the U-statistic. We derive non-asymptotic Gaussian approximation error bounds for the randomized incomplete U-statistics in high dimensions, namely in cases where the dimension is possibly much larger than the sample size, for both non-degenerate and degenerate kernels. In addition, we propose generic bootstrap methods for the incomplete U-statistics that are computationally much less demanding than existing bootstrap methods and establish finite sample validity of the proposed bootstrap methods. If time permits, I will also discuss the extension to infinite order U-statistics.
dimensional U-statistic. In the era of Big Data, the dimension of the U statistic and the sample size of the observations tend to be both large, and the computation of the U-statistic is prohibitively demanding. Data-dependent inferential procedures such as the empirical bootstrap for U-statistics is even more computationally expensive. To overcome such computational bottleneck, we introduce randomized incomplete U-statistics with sparse weights whose computational cost can be made independent of the order of the U-statistic. We derive non-asymptotic Gaussian approximation error bounds for the randomized incomplete U-statistics in high dimensions, namely in cases where the dimension is possibly much larger than the sample size, for both non-degenerate and degenerate kernels. In addition, we propose generic bootstrap methods for the incomplete U-statistics that are computationally much less demanding than existing bootstrap methods and establish finite sample validity of the proposed bootstrap methods. If time permits, I will also discuss the extension to infinite order U-statistics.
Explore Similar Events
-
Loading Similar Events...