Presented By: Department of Statistics
Statistics Department Seminar Series: Mohamad Kazem Shirani Faradonbeh, Postdoctoral Researcher, University of Florida
"Learning Unstable Time Series and Stabilizing Inputs"
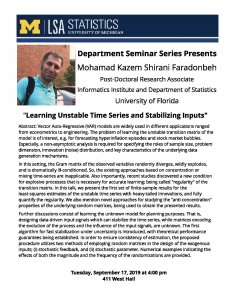
Abstract:
Vector Auto-Regressive (VAR) models are widely used in different applications ranged from econometrics to engineering. The problem of learning the unstable transition matrix of the model is of interest, e.g. for forecasting hyperinflation episodes and stock market bubbles. Especially, a non-asymptotic analysis is required for specifying the roles of sample size, problem dimension, innovation (noise) distribution, and key characteristics of the underlying data generation mechanisms.
In this setting, the Gram matrix of the observed variables randomly diverges, wildly explodes, and is dramatically ill-conditioned. So, the existing approaches based on concentration or mixing time-series are inapplicable. Also importantly, recent studies discovered a new condition for explosive processes that is necessary for accurate learning; being called "regularity" of the transition matrix. In this talk, we present the first set of finite-sample results for the least-squares estimates of the unstable time series with heavy-tailed innovations, and fully quantify the regularity. We also mention novel approaches for studying the "anti-concentration" properties of the underlying random matrices, being used to obtain the presented results.
Further discussions consist of learning the unknown model for planning purposes. That is, designing data-driven input signals which can stabilize the time series, while matrices encoding the evolution of the process and the influence of the input signals, are unknown. The first algorithm for fast stabilization under uncertainty is introduced, with theoretical performance guarantees being established. In order to ensure consistency of estimation, the proposed procedure utilizes two methods of employing random matrices in the design of the exogenous inputs; (i) stochastic feedback, and (ii) stochastic parameter. Numerical examples indicating the effects of both the magnitude and the frequency of the randomizations are provided.
Bio:
Mohamad Kazem Shirani Faradonbeh
is currently a Post-Doctoral Research Associate with the Informatics Institute and the Department of Statistics
at the University of Florida. He received the Ph.D. degree in Statistics from the University of Michigan in 2017, under the supervision of Ambuj Tewari and George Michailidis. Before that, he was an undergraduate student in the Department of Electrical Engineering, Sharif University of Technology. His research interests include sequential statistical analysis, reinforcement learning, data-driven intelligent tutoring, and control of network systems.
Vector Auto-Regressive (VAR) models are widely used in different applications ranged from econometrics to engineering. The problem of learning the unstable transition matrix of the model is of interest, e.g. for forecasting hyperinflation episodes and stock market bubbles. Especially, a non-asymptotic analysis is required for specifying the roles of sample size, problem dimension, innovation (noise) distribution, and key characteristics of the underlying data generation mechanisms.
In this setting, the Gram matrix of the observed variables randomly diverges, wildly explodes, and is dramatically ill-conditioned. So, the existing approaches based on concentration or mixing time-series are inapplicable. Also importantly, recent studies discovered a new condition for explosive processes that is necessary for accurate learning; being called "regularity" of the transition matrix. In this talk, we present the first set of finite-sample results for the least-squares estimates of the unstable time series with heavy-tailed innovations, and fully quantify the regularity. We also mention novel approaches for studying the "anti-concentration" properties of the underlying random matrices, being used to obtain the presented results.
Further discussions consist of learning the unknown model for planning purposes. That is, designing data-driven input signals which can stabilize the time series, while matrices encoding the evolution of the process and the influence of the input signals, are unknown. The first algorithm for fast stabilization under uncertainty is introduced, with theoretical performance guarantees being established. In order to ensure consistency of estimation, the proposed procedure utilizes two methods of employing random matrices in the design of the exogenous inputs; (i) stochastic feedback, and (ii) stochastic parameter. Numerical examples indicating the effects of both the magnitude and the frequency of the randomizations are provided.
Bio:
Mohamad Kazem Shirani Faradonbeh
is currently a Post-Doctoral Research Associate with the Informatics Institute and the Department of Statistics
at the University of Florida. He received the Ph.D. degree in Statistics from the University of Michigan in 2017, under the supervision of Ambuj Tewari and George Michailidis. Before that, he was an undergraduate student in the Department of Electrical Engineering, Sharif University of Technology. His research interests include sequential statistical analysis, reinforcement learning, data-driven intelligent tutoring, and control of network systems.
Explore Similar Events
-
Loading Similar Events...