Presented By: Industrial & Operations Engineering
Departmental Seminar (899): Eunhye Song, Pennsylvania State University
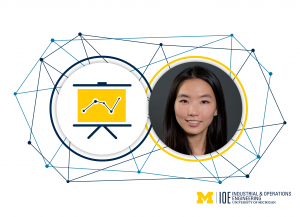
The Departmental Seminar Series is open to all. U-M Industrial and Operations Engineering graduate students and faculty are especially encouraged to attend.
The seminar will be followed by a reception in the IOE Commons (Room 1709) from 4 p.m. to 5 p.m.
Title: Sequential Bayesian risk set estimation for robust simulation optimization under input model uncertainty
Abstract:
This talk discusses a new way of approaching a discrete simulation optimization
problem when the input distributions of the simulation model are estimated with error from real-world observations. This problem is known as ‘simulation optimization under input uncertainty’ and has been studied actively in recent years. Most approaches provide either asymptotic guarantee that the selected solution is the real-world optimum as the real-world sample size increases or find the optimum to an alternative formulation such as the distributionally robust optimum. This work focuses on finite-sample inference on the relative performances of the solutions while uncertainty about the input models are captured by their Bayesian posteriors. A user-specified smallest optimality gap of interest is reflected to control conservativeness of the procedure, so that two solutions whose expected performances are within is considered practically indistinguishable. The -level risk set of solution is defined as the set of solutions whose expected performance is practically better () than with significant probability () under the posterior on the input models. The size of the risk set shows robustness of solution; an empty risk set implies that there is no practically better solution than even with input uncertainty. For efficient estimation of the risk set, the expected performance is modeled
as a Gaussian process (GP) that takes a solution and a collection of input distributions generated from their posterior as inputs. A one-step look-ahead sampling rule is proposed to choose which solution-distributions pair to simulate in the next iteration to minimize the estimation error of the risk set.
Bio:
Eunhye Song is the Harold and Inge Marcus Early Career Assistant Professor in Industrial and Manufacturing Engineering at Penn State University. She earned her PhD degree in Industrial Engineering and Management Sciences at Northwestern University in 2017 and MS and BS in Industrial and Systems Engineering at Korea Advanced Institute of Science and Technology (KAIST) in 2012 and 2010, respectively. Her research interests include design of simulation experiments, large-scale discrete simulation optimization, input uncertainty quantification, and simulation optimization in the presence of model risk. She has collaborated
with Simio, a leading discrete-event simulation software company, on developing a statistical tool to quantify input uncertainty for a Simio model, which is now a standard part of Simio’s software product. She also worked with General Motors’ R&D group on global sensitivity analysis of Vehicle Content Optimization simulator, which GM uses to find the optimal vehicle content portfolios of their major vehicle lines to maximize GM’s market share and profit. She is an active member of INFORMS Simulation Society (I-Sim) and currently serving on the I-Sim Diversity Committee chair.
The seminar will be followed by a reception in the IOE Commons (Room 1709) from 4 p.m. to 5 p.m.
Title: Sequential Bayesian risk set estimation for robust simulation optimization under input model uncertainty
Abstract:
This talk discusses a new way of approaching a discrete simulation optimization
problem when the input distributions of the simulation model are estimated with error from real-world observations. This problem is known as ‘simulation optimization under input uncertainty’ and has been studied actively in recent years. Most approaches provide either asymptotic guarantee that the selected solution is the real-world optimum as the real-world sample size increases or find the optimum to an alternative formulation such as the distributionally robust optimum. This work focuses on finite-sample inference on the relative performances of the solutions while uncertainty about the input models are captured by their Bayesian posteriors. A user-specified smallest optimality gap of interest is reflected to control conservativeness of the procedure, so that two solutions whose expected performances are within is considered practically indistinguishable. The -level risk set of solution is defined as the set of solutions whose expected performance is practically better () than with significant probability () under the posterior on the input models. The size of the risk set shows robustness of solution; an empty risk set implies that there is no practically better solution than even with input uncertainty. For efficient estimation of the risk set, the expected performance is modeled
as a Gaussian process (GP) that takes a solution and a collection of input distributions generated from their posterior as inputs. A one-step look-ahead sampling rule is proposed to choose which solution-distributions pair to simulate in the next iteration to minimize the estimation error of the risk set.
Bio:
Eunhye Song is the Harold and Inge Marcus Early Career Assistant Professor in Industrial and Manufacturing Engineering at Penn State University. She earned her PhD degree in Industrial Engineering and Management Sciences at Northwestern University in 2017 and MS and BS in Industrial and Systems Engineering at Korea Advanced Institute of Science and Technology (KAIST) in 2012 and 2010, respectively. Her research interests include design of simulation experiments, large-scale discrete simulation optimization, input uncertainty quantification, and simulation optimization in the presence of model risk. She has collaborated
with Simio, a leading discrete-event simulation software company, on developing a statistical tool to quantify input uncertainty for a Simio model, which is now a standard part of Simio’s software product. She also worked with General Motors’ R&D group on global sensitivity analysis of Vehicle Content Optimization simulator, which GM uses to find the optimal vehicle content portfolios of their major vehicle lines to maximize GM’s market share and profit. She is an active member of INFORMS Simulation Society (I-Sim) and currently serving on the I-Sim Diversity Committee chair.