Presented By: Department of Statistics
Livestream: Statistics Department Seminar Series: Tamara Broderick, Associate Professor, Department Electrical Engineering and Computer Science, Massachusetts Institute of Technology
"Fast Discovery of Pairwise Interactions in High Dimensions using Bayes"
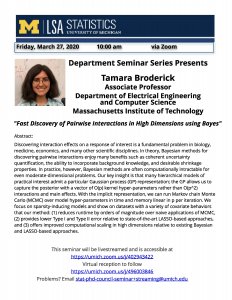
Abstract:
Discovering interaction effects on a response of interest is a fundamental problem in biology, medicine, economics, and many other scientific disciplines. In theory, Bayesian methods for discovering pairwise interactions enjoy many benefits such as coherent uncertainty quantification, the ability to incorporate background knowledge, and desirable shrinkage properties. In practice, however, Bayesian methods are often computationally intractable for even moderate-dimensional problems. Our key insight is that many hierarchical models of practical interest admit a particular Gaussian process (GP) representation; the GP allows us to capture the posterior with a vector of O(p) kernel hyper-parameters rather than O(p^2) interactions and main effects. With the implicit representation, we can run Markov chain Monte Carlo (MCMC) over model hyper-parameters in time and memory linear in p per iteration. We focus on sparsity-inducing models and show on datasets with a variety of covariate behaviors that our method: (1) reduces runtime by orders of magnitude over naive applications of MCMC, (2) provides lower Type I and Type II error relative to state-of-the-art LASSO-based approaches, and (3) offers improved computational scaling in high dimensions relative to existing Bayesian and LASSO-based approaches.
Livestream Info
This Seminar will be livestreamed using Zoom. When you click the meeting link on your PC or mobile device, it will direct you to the Zoom app (which you may wish to download in advance).
You will be able to see/hear the presenter, but your microphone/camera are off. If you’d like to ask a question at any time, please click the hand icon to “raise your hand” and a moderator can temporarily enable your microphone so you can ask a question and interact with the presenter. You can also use the Q&A feature to submit typed questions if you prefer.
Join from Computer/Tablet/Smartphone
Please click the link below to join the webinar:
https://umich.zoom.us/j/402943422
Join via Phone
iPhone one-tap :
US: +13126266799,,402943422# or +16468769923,,402943422#
Or Telephone:
Dial(for higher quality, dial a number based on your current location):
US: +1 312 626 6799 or +1 646 876 9923 or +1 346 248 7799 or +1 669 900 6833 or +1 253 215 8782 or +1 301 715 8592
Canada: +1 647 558 0588 or +1 778 907 2071 or +1 438 809 7799 or +1 587 328 1099 or +1 647 374 4685
Webinar ID: 402 943 422
International numbers available: https://umich.zoom.us/u/ac83BV4NUh
Virtual Reception:
Reception goes live several minutes after seminar concludes:
https://umich.zoom.us/j/496003846
If you encounter problems, you can email stat-phd-council-seminar+streaming@umich.edu and we will do our best to assist you.
Discovering interaction effects on a response of interest is a fundamental problem in biology, medicine, economics, and many other scientific disciplines. In theory, Bayesian methods for discovering pairwise interactions enjoy many benefits such as coherent uncertainty quantification, the ability to incorporate background knowledge, and desirable shrinkage properties. In practice, however, Bayesian methods are often computationally intractable for even moderate-dimensional problems. Our key insight is that many hierarchical models of practical interest admit a particular Gaussian process (GP) representation; the GP allows us to capture the posterior with a vector of O(p) kernel hyper-parameters rather than O(p^2) interactions and main effects. With the implicit representation, we can run Markov chain Monte Carlo (MCMC) over model hyper-parameters in time and memory linear in p per iteration. We focus on sparsity-inducing models and show on datasets with a variety of covariate behaviors that our method: (1) reduces runtime by orders of magnitude over naive applications of MCMC, (2) provides lower Type I and Type II error relative to state-of-the-art LASSO-based approaches, and (3) offers improved computational scaling in high dimensions relative to existing Bayesian and LASSO-based approaches.
Livestream Info
This Seminar will be livestreamed using Zoom. When you click the meeting link on your PC or mobile device, it will direct you to the Zoom app (which you may wish to download in advance).
You will be able to see/hear the presenter, but your microphone/camera are off. If you’d like to ask a question at any time, please click the hand icon to “raise your hand” and a moderator can temporarily enable your microphone so you can ask a question and interact with the presenter. You can also use the Q&A feature to submit typed questions if you prefer.
Join from Computer/Tablet/Smartphone
Please click the link below to join the webinar:
https://umich.zoom.us/j/402943422
Join via Phone
iPhone one-tap :
US: +13126266799,,402943422# or +16468769923,,402943422#
Or Telephone:
Dial(for higher quality, dial a number based on your current location):
US: +1 312 626 6799 or +1 646 876 9923 or +1 346 248 7799 or +1 669 900 6833 or +1 253 215 8782 or +1 301 715 8592
Canada: +1 647 558 0588 or +1 778 907 2071 or +1 438 809 7799 or +1 587 328 1099 or +1 647 374 4685
Webinar ID: 402 943 422
International numbers available: https://umich.zoom.us/u/ac83BV4NUh
Virtual Reception:
Reception goes live several minutes after seminar concludes:
https://umich.zoom.us/j/496003846
If you encounter problems, you can email stat-phd-council-seminar+streaming@umich.edu and we will do our best to assist you.
Explore Similar Events
-
Loading Similar Events...