Presented By: Interdisciplinary Seminar in Quantitative Methods (ISQM)
Interdisciplinary Seminar in Quantitative Methods (ISQM)
David Blei (Departments of Computer Science and Statistics at Columbia University)
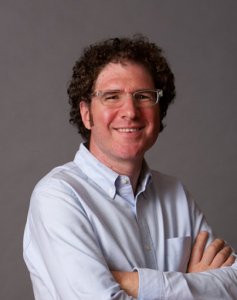
The Blessings of Multiple Causes (Joint with Yixin Wang)
ABSTRACT: Causal inference from observational data is a vital problem, but it comes with strong assumptions. Most methods require that we observe all confounders, variables that affect both the causal variables and the outcome variables. But whether we have observed all confounders is a famously untestable assumption. We describe the deconfounder, a way to do causal inference with weaker assumptions than the classical methods require.
How does the deconfounder work? While traditional causal methods measure the effect of a single cause on an outcome, many modern scientific studies involve multiple causes, different variables whose effects are simultaneously of interest. The deconfounder uses the correlation among multiple causes as evidence for unobserved confounders, combining unsupervised machine learning and predictive model checking to perform causal inference. We demonstrate the deconfounder on real-world data and simulation studies, and describe the theoretical requirements for the deconfounder to provide unbiased causal estimates.
David works in the fields of machine learning and Bayesian statistics.
The goal of the Interdisciplinary Seminar in Quantitative Methods is to provide an interdisciplinary environment where researchers can present and discuss cutting-edge research in quantitative methodology. The talks are aimed at a broad audience, with emphasis on conceptual rather than technical issues. The research presented is varied, ranging from new methodological developments to applied empirical papers that use methodology in an innovative way. We welcome speakers and audiences from all disciplines and fields, including the social, natural, biomedical, and behavioral sciences.
ABSTRACT: Causal inference from observational data is a vital problem, but it comes with strong assumptions. Most methods require that we observe all confounders, variables that affect both the causal variables and the outcome variables. But whether we have observed all confounders is a famously untestable assumption. We describe the deconfounder, a way to do causal inference with weaker assumptions than the classical methods require.
How does the deconfounder work? While traditional causal methods measure the effect of a single cause on an outcome, many modern scientific studies involve multiple causes, different variables whose effects are simultaneously of interest. The deconfounder uses the correlation among multiple causes as evidence for unobserved confounders, combining unsupervised machine learning and predictive model checking to perform causal inference. We demonstrate the deconfounder on real-world data and simulation studies, and describe the theoretical requirements for the deconfounder to provide unbiased causal estimates.
David works in the fields of machine learning and Bayesian statistics.
The goal of the Interdisciplinary Seminar in Quantitative Methods is to provide an interdisciplinary environment where researchers can present and discuss cutting-edge research in quantitative methodology. The talks are aimed at a broad audience, with emphasis on conceptual rather than technical issues. The research presented is varied, ranging from new methodological developments to applied empirical papers that use methodology in an innovative way. We welcome speakers and audiences from all disciplines and fields, including the social, natural, biomedical, and behavioral sciences.
Explore Similar Events
-
Loading Similar Events...