Presented By: Aerospace Engineering
AE585 Graduate Seminar: Distributed Control and Scalable Optimization for Large-scale Autonomy
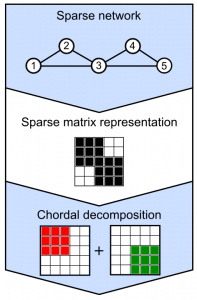
Yang Zheng
Postdoctoral Researcher
School of Engineering and Applied Sciences, Harvard University
Modern cyber-physical systems, such as drone formation, robot swarms, and transportation systems, can be of large scale and have sparse and distributed control logic due to limited information exchange. Many control and computational problems of practical interest remain unsolved due to the issues of non-convexity and complexity. The first part of this talk focuses on how to recover convexity for distributed control problems. I will present a new distributed control framework centered on the notion of sparsity invariance, which allows deriving convex approximation/reformulation of the largest known class of distributed control problems with sparsity constraints. I will show that the notion of sparsity invariance goes beyond the well-known notion of quadratic invariance. The second part of this talk focuses on the scalability of solving large-scale convex optimization problems. In particular, I will consider the class of semidefinite optimization problems. By exploiting the properties of chordal graphs and sparse positive semidefinite matrices, I will present a decomposition method that can scale sparse semidefinite optimization to large-scale instances, achieving massive scalability. The resulting algorithms have been implemented in the open-source solver: CDCS (Cone Decomposition Conic Solver). Extensions to a class of polynomial optimization, i.e., sparse sum-of-squares optimization, will be briefly discussed.
About the speaker:
Yang Zheng received the DPhil (Ph.D.) degree in Engineering Science from the University of Oxford, UK, in 2019. He received the B.E. and M.S. degrees from Tsinghua University, China, in 2013 and 2015, respectively. He is currently a postdoctoral researcher in the SEAS and CGBC at Harvard University. His research interests lie in the interface of learning, optimization, and control of network systems, and their applications to cyber-physical systems, especially autonomous vehicles and traffic systems. His work was acknowledged by several awards, including the Best Student Paper Award Finalist at the 2019 European Control Conference, the Best Student Paper Award at the 17th IEEE International Conference on Intelligent Transportation Systems, and the Best Paper Award at the 14th Intelligent Transportation Systems Asia-Pacific Forum. He is the recipient of the National Scholarship, Outstanding Graduate in Tsinghua University, and the Clarendon Scholarship at the University of Oxford. In 2018, he received the ABTA Doctoral Research Award in Engineering Science, and in 2019, he received the Chinese Government Award for Outstanding Self-financed Students Abroad.
Postdoctoral Researcher
School of Engineering and Applied Sciences, Harvard University
Modern cyber-physical systems, such as drone formation, robot swarms, and transportation systems, can be of large scale and have sparse and distributed control logic due to limited information exchange. Many control and computational problems of practical interest remain unsolved due to the issues of non-convexity and complexity. The first part of this talk focuses on how to recover convexity for distributed control problems. I will present a new distributed control framework centered on the notion of sparsity invariance, which allows deriving convex approximation/reformulation of the largest known class of distributed control problems with sparsity constraints. I will show that the notion of sparsity invariance goes beyond the well-known notion of quadratic invariance. The second part of this talk focuses on the scalability of solving large-scale convex optimization problems. In particular, I will consider the class of semidefinite optimization problems. By exploiting the properties of chordal graphs and sparse positive semidefinite matrices, I will present a decomposition method that can scale sparse semidefinite optimization to large-scale instances, achieving massive scalability. The resulting algorithms have been implemented in the open-source solver: CDCS (Cone Decomposition Conic Solver). Extensions to a class of polynomial optimization, i.e., sparse sum-of-squares optimization, will be briefly discussed.
About the speaker:
Yang Zheng received the DPhil (Ph.D.) degree in Engineering Science from the University of Oxford, UK, in 2019. He received the B.E. and M.S. degrees from Tsinghua University, China, in 2013 and 2015, respectively. He is currently a postdoctoral researcher in the SEAS and CGBC at Harvard University. His research interests lie in the interface of learning, optimization, and control of network systems, and their applications to cyber-physical systems, especially autonomous vehicles and traffic systems. His work was acknowledged by several awards, including the Best Student Paper Award Finalist at the 2019 European Control Conference, the Best Student Paper Award at the 17th IEEE International Conference on Intelligent Transportation Systems, and the Best Paper Award at the 14th Intelligent Transportation Systems Asia-Pacific Forum. He is the recipient of the National Scholarship, Outstanding Graduate in Tsinghua University, and the Clarendon Scholarship at the University of Oxford. In 2018, he received the ABTA Doctoral Research Award in Engineering Science, and in 2019, he received the Chinese Government Award for Outstanding Self-financed Students Abroad.
Explore Similar Events
-
Loading Similar Events...