Presented By: Aerospace Engineering
Defense Dissertation: Variational and Time-Distributed Methods for Real-time Model Predictive Control
Dominic Liao-McPherson
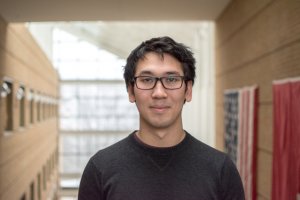
Dominic Liao-McPherson
Committee:
Prof. Ilya Kolmanovsky (Chair)
Prof. Jing Sun (Cognate)
Prof. Alex Gorodetsky
Dr. Ken Butts (External, Toyota)
Time/location:
March 19th 2020, 10:00 AM
GM Conference Room, Lurie Engineering Center (4th floor)
Autonomous systems are beginning to impact many aspects of society, e.g., drones are becoming commonplace and self-driving cars are being developed on an industrial scale. These systems need to make complex decisions onboard; mathematical optimization is a powerful paradigm for approaching these problems. In particular, Model Predictive Control (MPC), a powerful optimization-based methodology for controlling constrained systems, is an important enabler for autonomy. However, solving optimization problems reliably, in real-time, using limited onboard computing resources, is a difficult undertaking.
This dissertation addresses the development, implementation, and validation of several numerical methods for solving optimal control problems (OCPs) in real-time. First, I present FBstab, a novel quadratic programming algorithm with strong robustness properties that is easy to warmstart and can exploit the structure of optimal control problems. Second, I introduce Time-distributed Optimization (TDO), a unifying framework for studying the system theoretic consequences of computation limits, which I use to show that, in some situations, it is possible to recover the stability and robustness properties of optimal MPC despite limited resources. Finally, I illustrate the applicability of these methods in the real-world through a diesel engine control example.
Committee:
Prof. Ilya Kolmanovsky (Chair)
Prof. Jing Sun (Cognate)
Prof. Alex Gorodetsky
Dr. Ken Butts (External, Toyota)
Time/location:
March 19th 2020, 10:00 AM
GM Conference Room, Lurie Engineering Center (4th floor)
Autonomous systems are beginning to impact many aspects of society, e.g., drones are becoming commonplace and self-driving cars are being developed on an industrial scale. These systems need to make complex decisions onboard; mathematical optimization is a powerful paradigm for approaching these problems. In particular, Model Predictive Control (MPC), a powerful optimization-based methodology for controlling constrained systems, is an important enabler for autonomy. However, solving optimization problems reliably, in real-time, using limited onboard computing resources, is a difficult undertaking.
This dissertation addresses the development, implementation, and validation of several numerical methods for solving optimal control problems (OCPs) in real-time. First, I present FBstab, a novel quadratic programming algorithm with strong robustness properties that is easy to warmstart and can exploit the structure of optimal control problems. Second, I introduce Time-distributed Optimization (TDO), a unifying framework for studying the system theoretic consequences of computation limits, which I use to show that, in some situations, it is possible to recover the stability and robustness properties of optimal MPC despite limited resources. Finally, I illustrate the applicability of these methods in the real-world through a diesel engine control example.