Presented By: U-M Industrial & Operations Engineering
Departmental Seminar (899): Cong Shi, U-M IOE
Network Revenue Management with Online Inverse Batch Gradient Descent Method
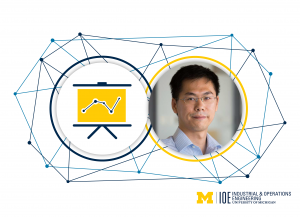
The Departmental Seminar Series is open to all. U-M Industrial and Operations Engineering graduate students and faculty are especially encouraged to attend.
Title:
Network Revenue Management with Online Inverse Batch Gradient Descent Method
Abstract:
We consider a general class of price-based network revenue management problems that a firm aims to maximize revenue from multiple products produced with multiple types of resources endowed with limited inventory over a finite selling season. A salient feature of our problem is that the firm does not know the underlying demand function that maps prices to demand rate, which must be learned from sales data. It is well known that for almost all classes of demand functions, the revenue rate function is not concave in the products' prices but is concave in products' market shares (or price-controlled demand rates). This creates challenges in adopting any stochastic gradient descent based methods in the price space. We propose a novel nonparametric learning algorithm termed online inverse batch gradient descent (IGD) algorithm. For the large scale systems wherein all resources' inventories and the length of the horizon are proportionally scaled by a parameter $k$, we establish a dimension-independent regret bound of $O( k^{4/5} \log k)$. This result is independent of the number of products and resources and works for a continuum action-set prices and the demand functions that are only once differentiable. Our result guarantees the efficacy of both algorithms in the high dimensional systems where the number of products or resources is large and the prices are continuous. (This is a joint work with Dr. Yiwei Chen.)
Bio:
Cong Shi is an associate professor in the Department of Industrial and Operations Engineering at the University of Michigan at Ann Arbor. His main research interests include supply chain management, revenue management, and service operations. He has won the first place in the INFORMS George Nicholson Student Paper Competition, the third place in the INFORMS Junior Faculty Interest Group (JFIG) Paper Competition, and the finalist for the MSOM Data Driven Challenge. He received his Ph.D. in Operations Research from MIT in 2012, and his B.S. in Mathematics from the National University of Singapore in 2007.
Title:
Network Revenue Management with Online Inverse Batch Gradient Descent Method
Abstract:
We consider a general class of price-based network revenue management problems that a firm aims to maximize revenue from multiple products produced with multiple types of resources endowed with limited inventory over a finite selling season. A salient feature of our problem is that the firm does not know the underlying demand function that maps prices to demand rate, which must be learned from sales data. It is well known that for almost all classes of demand functions, the revenue rate function is not concave in the products' prices but is concave in products' market shares (or price-controlled demand rates). This creates challenges in adopting any stochastic gradient descent based methods in the price space. We propose a novel nonparametric learning algorithm termed online inverse batch gradient descent (IGD) algorithm. For the large scale systems wherein all resources' inventories and the length of the horizon are proportionally scaled by a parameter $k$, we establish a dimension-independent regret bound of $O( k^{4/5} \log k)$. This result is independent of the number of products and resources and works for a continuum action-set prices and the demand functions that are only once differentiable. Our result guarantees the efficacy of both algorithms in the high dimensional systems where the number of products or resources is large and the prices are continuous. (This is a joint work with Dr. Yiwei Chen.)
Bio:
Cong Shi is an associate professor in the Department of Industrial and Operations Engineering at the University of Michigan at Ann Arbor. His main research interests include supply chain management, revenue management, and service operations. He has won the first place in the INFORMS George Nicholson Student Paper Competition, the third place in the INFORMS Junior Faculty Interest Group (JFIG) Paper Competition, and the finalist for the MSOM Data Driven Challenge. He received his Ph.D. in Operations Research from MIT in 2012, and his B.S. in Mathematics from the National University of Singapore in 2007.
Explore Similar Events
-
Loading Similar Events...