Presented By: Michigan Robotics
Deformable Object Manipulation: Learning While Doing
Robotics PhD Defense, Dale McConachie
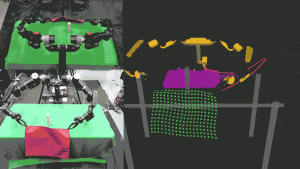
This dissertation is motivated by two research questions: (1) How can robots perform a broad range of useful tasks with deformable objects without a time consuming modelling or data collection phase? and (2) How can robots take advantage of information learned while manipulating deformable objects?
To address the first question, I propose a framework for deformable object manipulation that interleaves planning and control, enabling complex manipulation tasks without relying on high-fidelity modeling or simulation. Each part of the framework uses a different representation of the deformable object that is well suited for the specific requirements of each component. The key idea behind these techniques is that we do not need to explicitly model and control every part of the deformable object, instead relying on the object's natural compliance in many situations.
For the second question, I consider the two major components of my framework and examine what can cause failure in each. The goal then is to learn from experience gathered while performing tasks in order to avoid making the same mistake again and again. To this end I formulate the controller's task as a Multi-Armed Bandit problem, enabling the controller to chose models based on the current circumstances. For the planner, I present a method to learn when we can rely on the robot's model of the deformable object, enabling the planner to avoid generating plans that are infeasible.
This framework is demonstrated in simulation with free floating grippers as well as on a 16 DoF physical robot, where reachability and dual-arm constraints make the tasks more difficult.
Dale's research interest include machine learning, controls, and motion planning. He is currently working on applying ideas from these fields to deformable object manipulation and related topics. In particular Dale is interested in algorithms that enable robots to perform tasks such as folding clothes, or making a bed, that are easy for humans to master, but are extremely challenging for robots. Dale graduated from Carnegie Mellon University with both college and university honors, and is also the recipient of the Frank J. Marshall Scholar Award.
To address the first question, I propose a framework for deformable object manipulation that interleaves planning and control, enabling complex manipulation tasks without relying on high-fidelity modeling or simulation. Each part of the framework uses a different representation of the deformable object that is well suited for the specific requirements of each component. The key idea behind these techniques is that we do not need to explicitly model and control every part of the deformable object, instead relying on the object's natural compliance in many situations.
For the second question, I consider the two major components of my framework and examine what can cause failure in each. The goal then is to learn from experience gathered while performing tasks in order to avoid making the same mistake again and again. To this end I formulate the controller's task as a Multi-Armed Bandit problem, enabling the controller to chose models based on the current circumstances. For the planner, I present a method to learn when we can rely on the robot's model of the deformable object, enabling the planner to avoid generating plans that are infeasible.
This framework is demonstrated in simulation with free floating grippers as well as on a 16 DoF physical robot, where reachability and dual-arm constraints make the tasks more difficult.
Dale's research interest include machine learning, controls, and motion planning. He is currently working on applying ideas from these fields to deformable object manipulation and related topics. In particular Dale is interested in algorithms that enable robots to perform tasks such as folding clothes, or making a bed, that are easy for humans to master, but are extremely challenging for robots. Dale graduated from Carnegie Mellon University with both college and university honors, and is also the recipient of the Frank J. Marshall Scholar Award.
Explore Similar Events
-
Loading Similar Events...