Presented By: Department of Statistics Seminar Series
Statistics Department Seminar Series: Michael Rosenblum, Associate Professor of Biostatistics, Johns Hopkins Bloomberg School of Public Health
“Improving Precision and Power in Randomized Trials for COVID-19 Treatments Using Covariate Adjustment, for Binary, Ordinal, or Time to Event Outcomes”
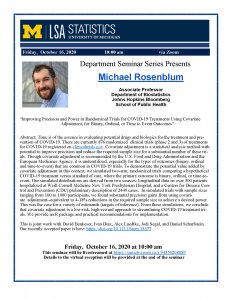
Abstract: Time is of the essence in evaluating potential drugs and biologics for the treatment and prevention of COVID-19. There are currently 876 randomized clinical trials (phase 2 and 3) of treatments for COVID-19 registered on clinicaltrials.gov. Covariate adjustment is a statistical analysis method with potential to improve precision and reduce the required sample size for a substantial number of these trials. Though covariate adjustment is recommended by the U.S. Food and Drug Administration and the European Medicines Agency, it is underutilized, especially for the types of outcomes (binary, ordinal and time-to-event) that are common in COVID-19 trials. To demonstrate the potential value added by covariate adjustment in this context, we simulated two-arm, randomized trials comparing a hypothetical COVID-19 treatment versus standard of care, where the primary outcome is binary, ordinal, or time-to-event. Our simulated distributions are derived from two sources: longitudinal data on over 500 patients hospitalized at Weill Cornell Medicine New York Presbyterian Hospital, and a Centers for Disease Control and Prevention (CDC) preliminary description of 2449 cases. In simulated trials with sample sizes ranging from 100 to 1000 participants, we found substantial precision gains from using covariate adjustment--equivalent to 4-18% reductions in the required sample size to achieve a desired power. This was the case for a variety of estimands (targets of inference). From these simulations, we conclude that covariate adjustment is a low-risk, high-reward approach to streamlining COVID-19 treatment trials. We provide an R package and practical recommendations for implementation.
This is joint work with David Benkeser, Ivan Diaz, Alex Luedtke, Jodi Segal, and Daniel Scharfstein. Our recently accepted paper is here: https://doi.org/10.1111/biom.13377
This seminar will be livestreamed via Zoom https://umich.zoom.us/j/94350208889
There will be a virtual reception to follow.
This is joint work with David Benkeser, Ivan Diaz, Alex Luedtke, Jodi Segal, and Daniel Scharfstein. Our recently accepted paper is here: https://doi.org/10.1111/biom.13377
This seminar will be livestreamed via Zoom https://umich.zoom.us/j/94350208889
There will be a virtual reception to follow.
Explore Similar Events
-
Loading Similar Events...