Presented By: Industrial & Operations Engineering
SEMINAR: "Data-Driven Sample-Average Approximation for Stochastic Optimization with Covariate Information" — Jim Luedtke
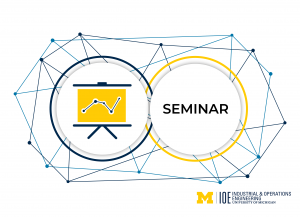
The Departmental Seminar Series is open to all. U-M Industrial and Operations Engineering graduate students and faculty are especially encouraged to attend.
Title:
Data-Driven Sample-Average Approximation for Stochastic Optimization with Covariate Information
Abstract:
We consider optimization models for decision-making in which parameters within the optimization model are uncertain, but predictions of these parameters can be made using available covariate information. We consider a data-driven setting in which we have observations of the uncertain parameters together with concurrently-observed covariates. Given a new covariate observation, the goal is to choose a decision that minimizes the expected cost conditioned on this observation. We investigate a data-driven framework in which the outputs from a machine learning prediction model are directly used to define a stochastic programming sample average approximation (SAA). The framework is flexible and accommodates parametric, nonparametric, and semiparametric regression techniques. The basic version of this framework is not new, but we are the first to analyze the procedure and derive conditions on the data generation process, the prediction model, and the stochastic program under which solutions of these data-driven SAAs are consistent and asymptotically optimal. We also derive convergence rates and finite sample guarantees. We also propose new variations that use out-of-sample residuals of leave-one-out prediction models for scenario generation. Computational experiments validate our theoretical results, demonstrate the potential advantages of our data-driven formulations over existing approaches (even when the prediction model is misspecified), and illustrate the benefits of our new variants in the limited data regime.
Bio:
Jim Luedtke is a Professor and Associate Chair for Graduate Studies in the department of Industrial and Systems Engineering at the University of Wisconsin-Madison. Luedtke earned his PhD at Georgia Tech and did postdoctoral work at the IBM T.J. Watson Research Center. Luedtke’s research is focused on methods for solving stochastic and mixed-integer optimization problems, as well as applications of such models. Luedtke is a recipient of an NSF CAREER award, was a finalist in the INFORMS JFIG Best Paper competition, and was awarded the INFORMS Optimization Society Prize for Young Researchers. Luedtke serves on the editorial boards of the journals SIAM Journal on Optimization and Mathematical Programming Computation, is the current secretary of the SIAM Activity Group in Optimization, and is chair of the Mathematical Optimization Society Publications Committee.
Title:
Data-Driven Sample-Average Approximation for Stochastic Optimization with Covariate Information
Abstract:
We consider optimization models for decision-making in which parameters within the optimization model are uncertain, but predictions of these parameters can be made using available covariate information. We consider a data-driven setting in which we have observations of the uncertain parameters together with concurrently-observed covariates. Given a new covariate observation, the goal is to choose a decision that minimizes the expected cost conditioned on this observation. We investigate a data-driven framework in which the outputs from a machine learning prediction model are directly used to define a stochastic programming sample average approximation (SAA). The framework is flexible and accommodates parametric, nonparametric, and semiparametric regression techniques. The basic version of this framework is not new, but we are the first to analyze the procedure and derive conditions on the data generation process, the prediction model, and the stochastic program under which solutions of these data-driven SAAs are consistent and asymptotically optimal. We also derive convergence rates and finite sample guarantees. We also propose new variations that use out-of-sample residuals of leave-one-out prediction models for scenario generation. Computational experiments validate our theoretical results, demonstrate the potential advantages of our data-driven formulations over existing approaches (even when the prediction model is misspecified), and illustrate the benefits of our new variants in the limited data regime.
Bio:
Jim Luedtke is a Professor and Associate Chair for Graduate Studies in the department of Industrial and Systems Engineering at the University of Wisconsin-Madison. Luedtke earned his PhD at Georgia Tech and did postdoctoral work at the IBM T.J. Watson Research Center. Luedtke’s research is focused on methods for solving stochastic and mixed-integer optimization problems, as well as applications of such models. Luedtke is a recipient of an NSF CAREER award, was a finalist in the INFORMS JFIG Best Paper competition, and was awarded the INFORMS Optimization Society Prize for Young Researchers. Luedtke serves on the editorial boards of the journals SIAM Journal on Optimization and Mathematical Programming Computation, is the current secretary of the SIAM Activity Group in Optimization, and is chair of the Mathematical Optimization Society Publications Committee.
Explore Similar Events
-
Loading Similar Events...