Presented By: Climate and Space Sciences and Engineering
CLASP Dissertation Defense: Rajeswari Balasubramaniam
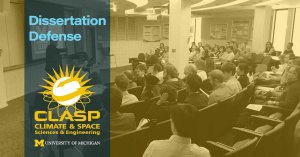
Rajeswari Balasubramaniam will be defending her PhD thesis via Zoom: https://umich.zoom.us/j/96764825341
Meeting ID: 967 6482 5341
Passcode: 725995.
TITLE: Investigating the Sensitivity of Spaceborne GNSS-R Measurements to Ocean Surface Winds and Rain
Faculty Advisor: Chris Ruf
ABSTRACT: Earth remote sensing using reflected Global Navigation Satellite System (GNSS) signals is an emerging trend, especially for ocean surface wind measurements, primarily due to its all day/all-weather capability. GNSS-Reflectometry (GNSS-R) measurements of ocean surface scattering cross section are directly related to the surface roughness at scale sizes ranging from small capillary waves to long gravity waves. These roughness scales are predominantly due to swell, surface winds and other meteorological phenomena such as rain. In this study we are interested in understanding and characterizing the impact of these phenomena on GNSS-R signals through observations and models in order to develop a better understanding of the geophysical parameters retrieved from these measurements.
In the first part of this work, we look at GNSS-R measurements made by the NASA Cyclone Global Navigation Satellite System (CYGNSS) for developing an effective wind retrieval model function for GNSS-R measurements. In a fully developed sea state, the wind field has a constant speed and direction over a sufficiently long time and distance and can be assumed to be spatially homogeneous; thus the surface roughness at all scale sizes can be assumed to be wind driven. In this case, a single Fully Developed Seas (FDS) Geophysical Model Function (GMF) is constructed which relates the scattering cross section to the near surface wind speed. However, the sea age and fetch length conditions inside a hurricane are in general not consistent with a fully developed sea state. Therefore, a separate empirical Young Sea Limited Fetch (YSLF) GMF is developed to represent the conditions inside a hurricane. Also, the degree of under development of the seas is not constant inside hurricanes and conditions vary significantly with azimuthal location within the hurricane due to changes in the relative alignment of the storm’s forward motion and its cyclonic rotation. As a result, the relationship between the surface roughness at different scale sizes is azimuthally dependent, as is the relationship between scattering cross section and wind speed represented by the GMF. The azimuthal dependence of the scattering cross-section is modelled and a modified azimuthal YSLF GMF is constructed using measurements by CYGNSS which are nearly coincident with HWRF reanalysis winds for 19 hurricanes in 2017 and 2018.
Next, we study the impact of rain on CYGNSS measurements. GNSS-R uses L-band navigation signals that can penetrate through clouds and rain. Rain has a negligible impact on the transmitted signal in terms of path attenuation at this wavelength. However, there are other effects due to rain, such as changes in surface roughness and rain induced local winds, which can significantly alter the measurements. Currently there are no careful observation-based characterizations of the impact of rain on radar forward scatter, which is the nature of operation of GNSS-R missions. In this part of the study we propose a 3-fold rain model for GNSS-R signals which accounts for: 1) attenuation; 2) surface effects of rain; and 3) rain induced local winds. The attenuation model suggests that a total of 96% or greater transmissivity exists at L-Band up to a rain rate of 30mm/hr. A perturbation model is used to characterize the other two rain effects. It suggests that rain is accompanied by an overall reduction in the scattering cross-section of the ocean surface and, most importantly, this effect is observed only up to surface wind speeds of ~15 m/s, beyond which the gravity capillary waves dominate the scattering in the quasi-specular direction. This work binds together several rain-related phenomena and enhances our overall understanding of rain effects on GNSS-R measurements.
Next, we try to understand the behavior of GNSS-R signals in a near specular scattering regime (surface wind speeds < 5 m/s). Over the ocean surface, roughness can be described by the Mean Square Slope (MSS) which is the integration of the omnidirectional wave spectrum up to an upper limit on the wavenumber which is defined by the Geometric Optics (GO) approximation. In this work we have identified that this upper limit is not applicable at very low wind speed ranges. A new wavenumber model is proposed that shows a dependence of the upper limit on wind speed at very low wind speed ranges (< 5 m/s) and asymptotes to the GO limit at higher wind speeds. This model suggests that the GO limit breaks down at very low wind speeds, and it is under those conditions that the effects of surface roughening by rain becomes significant and is measurable due to an increase in the upper bound on wavenumbers that affect the MSS.
Finally, one of the important objectives for the CYGNSS mission is to provide high quality global scale GNSS-R measurements that can reliably be used for ocean science applications such as the study of ocean wind speed dynamics, tropical cyclone genesis, coupled ocean wave modelling and assimilation into Numerical Weather Prediction models. To achieve this goal, strong quality control filters are needed to detect and remove outlier measurements. Currently, quality control of CYGNSS data products are based on fixed thresholds on various engineering, instrument, and measurement conditions. In this part of the work we develop a Neural Network based quality control filter for automated outlier detection of CYGNSS retrieved winds. The primary merit of the proposed Machine Learning (ML) filter is its ability to better account for interactions between the individual engineering, instrument and measurement conditions than can separate thresholded flags for each one. Use of ML capabilities to capture inherent patterns in the data provides an efficient and effective mechanism to detect and remove outlier measurements, thereby significantly improving the data quality.
Please plan to attend virtually.
Meeting ID: 967 6482 5341
Passcode: 725995.
TITLE: Investigating the Sensitivity of Spaceborne GNSS-R Measurements to Ocean Surface Winds and Rain
Faculty Advisor: Chris Ruf
ABSTRACT: Earth remote sensing using reflected Global Navigation Satellite System (GNSS) signals is an emerging trend, especially for ocean surface wind measurements, primarily due to its all day/all-weather capability. GNSS-Reflectometry (GNSS-R) measurements of ocean surface scattering cross section are directly related to the surface roughness at scale sizes ranging from small capillary waves to long gravity waves. These roughness scales are predominantly due to swell, surface winds and other meteorological phenomena such as rain. In this study we are interested in understanding and characterizing the impact of these phenomena on GNSS-R signals through observations and models in order to develop a better understanding of the geophysical parameters retrieved from these measurements.
In the first part of this work, we look at GNSS-R measurements made by the NASA Cyclone Global Navigation Satellite System (CYGNSS) for developing an effective wind retrieval model function for GNSS-R measurements. In a fully developed sea state, the wind field has a constant speed and direction over a sufficiently long time and distance and can be assumed to be spatially homogeneous; thus the surface roughness at all scale sizes can be assumed to be wind driven. In this case, a single Fully Developed Seas (FDS) Geophysical Model Function (GMF) is constructed which relates the scattering cross section to the near surface wind speed. However, the sea age and fetch length conditions inside a hurricane are in general not consistent with a fully developed sea state. Therefore, a separate empirical Young Sea Limited Fetch (YSLF) GMF is developed to represent the conditions inside a hurricane. Also, the degree of under development of the seas is not constant inside hurricanes and conditions vary significantly with azimuthal location within the hurricane due to changes in the relative alignment of the storm’s forward motion and its cyclonic rotation. As a result, the relationship between the surface roughness at different scale sizes is azimuthally dependent, as is the relationship between scattering cross section and wind speed represented by the GMF. The azimuthal dependence of the scattering cross-section is modelled and a modified azimuthal YSLF GMF is constructed using measurements by CYGNSS which are nearly coincident with HWRF reanalysis winds for 19 hurricanes in 2017 and 2018.
Next, we study the impact of rain on CYGNSS measurements. GNSS-R uses L-band navigation signals that can penetrate through clouds and rain. Rain has a negligible impact on the transmitted signal in terms of path attenuation at this wavelength. However, there are other effects due to rain, such as changes in surface roughness and rain induced local winds, which can significantly alter the measurements. Currently there are no careful observation-based characterizations of the impact of rain on radar forward scatter, which is the nature of operation of GNSS-R missions. In this part of the study we propose a 3-fold rain model for GNSS-R signals which accounts for: 1) attenuation; 2) surface effects of rain; and 3) rain induced local winds. The attenuation model suggests that a total of 96% or greater transmissivity exists at L-Band up to a rain rate of 30mm/hr. A perturbation model is used to characterize the other two rain effects. It suggests that rain is accompanied by an overall reduction in the scattering cross-section of the ocean surface and, most importantly, this effect is observed only up to surface wind speeds of ~15 m/s, beyond which the gravity capillary waves dominate the scattering in the quasi-specular direction. This work binds together several rain-related phenomena and enhances our overall understanding of rain effects on GNSS-R measurements.
Next, we try to understand the behavior of GNSS-R signals in a near specular scattering regime (surface wind speeds < 5 m/s). Over the ocean surface, roughness can be described by the Mean Square Slope (MSS) which is the integration of the omnidirectional wave spectrum up to an upper limit on the wavenumber which is defined by the Geometric Optics (GO) approximation. In this work we have identified that this upper limit is not applicable at very low wind speed ranges. A new wavenumber model is proposed that shows a dependence of the upper limit on wind speed at very low wind speed ranges (< 5 m/s) and asymptotes to the GO limit at higher wind speeds. This model suggests that the GO limit breaks down at very low wind speeds, and it is under those conditions that the effects of surface roughening by rain becomes significant and is measurable due to an increase in the upper bound on wavenumbers that affect the MSS.
Finally, one of the important objectives for the CYGNSS mission is to provide high quality global scale GNSS-R measurements that can reliably be used for ocean science applications such as the study of ocean wind speed dynamics, tropical cyclone genesis, coupled ocean wave modelling and assimilation into Numerical Weather Prediction models. To achieve this goal, strong quality control filters are needed to detect and remove outlier measurements. Currently, quality control of CYGNSS data products are based on fixed thresholds on various engineering, instrument, and measurement conditions. In this part of the work we develop a Neural Network based quality control filter for automated outlier detection of CYGNSS retrieved winds. The primary merit of the proposed Machine Learning (ML) filter is its ability to better account for interactions between the individual engineering, instrument and measurement conditions than can separate thresholded flags for each one. Use of ML capabilities to capture inherent patterns in the data provides an efficient and effective mechanism to detect and remove outlier measurements, thereby significantly improving the data quality.
Please plan to attend virtually.
Explore Similar Events
-
Loading Similar Events...