Presented By: Weinberg Institute for Cognitive Science
Reinforcement Learning for Sparse-Reward Object-Interaction Tasks in a First-person Simulated 3D Environment
A talk by Wilka Carvalho
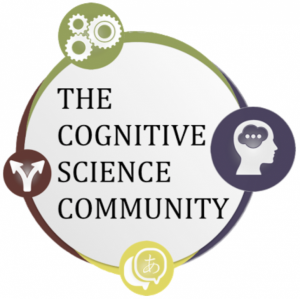
First-person object-interaction tasks in high-fidelity, 3D, simulated environments such as the AI2Thor virtual home-environment pose significant sample-efficiency challenges for reinforcement learning (RL) agents learning from sparse task rewards. To alleviate these challenges, prior work has provided extensive supervision via a combination of reward-shaping, ground-truth object-information, and expert demonstrations. In this work, we show that one can learn object-interaction tasks from scratch without supervision by learning an attentive object-model as an auxiliary task during task learning with an object-centric relational RL agent. Our key insight is that learning an object-model that incorporates object-relationships into forward prediction provides a dense learning signal for unsupervised representation learning of both objects and their relationships. This, in turn, enables faster policy learning for an object-centric relational RL agent. We demonstrate our agent by introducing a set of challenging object-interaction tasks in the AI2Thor environment where learning with our attentive object-model is key to strong performance. Specifically, by comparing our agent and relational RL agents with alternative auxiliary tasks with a relational RL agent equipped with ground-truth object-information, we find that learning with our object-model best closes the performance gap in terms of both learning speed and maximum success rate. Additionally, we find that incorporating object-relationships into an object-model's forward predictions is key to learning representations that capture object-category and object-state.
Zoom link: https://umich.zoom.us/j/99927591266
Meeting ID: 999 2759 1266
Password: cogsci
Wilka Carvalho is currently a PhD student studying machine learning at UM. Check out his website for research publications, experiences, helpful resources in machine learning or pursuing grad/PhD, and ways to reach out.
Zoom link: https://umich.zoom.us/j/99927591266
Meeting ID: 999 2759 1266
Password: cogsci
Wilka Carvalho is currently a PhD student studying machine learning at UM. Check out his website for research publications, experiences, helpful resources in machine learning or pursuing grad/PhD, and ways to reach out.
Livestream Information
ZoomNovember 5, 2020 (Thursday) 5:00pm
Meeting ID: 99927591266
Meeting Password: cogsci
Explore Similar Events
-
Loading Similar Events...