Presented By: Michigan Institute for Computational Discovery and Engineering
Tianle Yuan: Artificial Intelligence-based Cloud Distributor (AI-CD): Modeling Clouds at Different Scales
MICDE Seminar Series
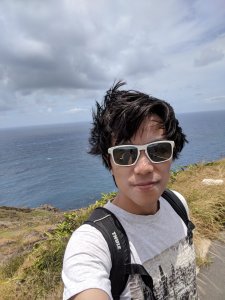
Abstract: Here we introduce the artificial intelligence-based cloud distributor (AI-CD) approach to generate cloud fields across different scales and cloud types. We show that generative adversarial nets (GANs) can not only generate realistic cloud fields with corresponding meteorological variables, but also capture known physical relationship between cloud fields and meteorological variables such as sea surface temperature, atmospheric stability, and relative humidity etc. We demonstrate that this approach works across a large range of spatial scales: from individual grid points (sub-grid process modeling), multiple grids, to global scale. In addition, the AI-CD approach is stochastic in nature. We suggest the AI-CD approach can be used as a data-drive framework for stochastic cloud parameterization.
Bio: Dr. Yuan got his B.S. in Geophysics and Computer Science in Peking Univ., PhD from University of Maryland, College Park, in 2008. After graduation, he is affiliated with Joint Center for Earth Systems Technologies (JCET) at the UMBC and NASA GSFC as an Associated Research Scientist. His research interest includes cloud and aerosol climate feedback, aerosol-cloud interactions, remote sensing, cloud physics, and application of ML/Deep Learning in Earth science. In deep learning applications, Dr. Yuan published a few papers in modeling sub-grid clouds, global scale clouds, hurricane prediction, finding ship-tracks, and supervised and unsupervised cloud morphology classifications.
Bio: Dr. Yuan got his B.S. in Geophysics and Computer Science in Peking Univ., PhD from University of Maryland, College Park, in 2008. After graduation, he is affiliated with Joint Center for Earth Systems Technologies (JCET) at the UMBC and NASA GSFC as an Associated Research Scientist. His research interest includes cloud and aerosol climate feedback, aerosol-cloud interactions, remote sensing, cloud physics, and application of ML/Deep Learning in Earth science. In deep learning applications, Dr. Yuan published a few papers in modeling sub-grid clouds, global scale clouds, hurricane prediction, finding ship-tracks, and supervised and unsupervised cloud morphology classifications.
Explore Similar Events
-
Loading Similar Events...