Presented By: Department of Statistics
Statistics Department Seminar Series: Xiaotong Shen, John Black Johnston Distinguished Professor, School of Statistics, University of Minnesota
"Inference for a directed acyclic graphical model with interventions"
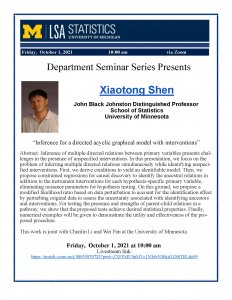
Abstract: Inference of multiple directed relations between primary variables presents challenges in the presence of unspecified interventions. In this presentation, we focus on the problem of inferring multiple directed relations simultaneously while identifying unspecified interventions. First, we derive conditions to yield an identifiable model. Then, we propose constrained regressions for causal discovery to identify the ancestral relations in addition to the instrument interventions for each hypothesis-specific primary variable, eliminating nuisance parameters for hypothesis testing. On this ground, we propose a modified likelihood ratio based on data perturbation to account for the identification effect by perturbing original data to assess the uncertainty associated with identifying ancestors and interventions. For testing the presence and strengths of parent-child relations in a pathway, we show that the proposed tests achieve desired statistical properties. Finally, numerical examples will be given to demonstrate the utility and effectiveness of the proposed procedure.
This work is joint with Chunlin Li and Wei Pan at the University of Minnesota.
Xiaotong T. Shen is the John Black Johnston Distinguished Professor in the College of Liberal Arts at the University of Minnesota. His areas of interest include machine learning and data mining, high-dimensional analysis, graphical models, large margin methods, personalization, recommender systems, natural language processing and text mining, and nonconvex minimization. His current research effort is devoted to the further development of structured learning, collabrative learning, and scalable analysis. The targeted application areas are biomedical sciences, artificial intelligence, and engineering.
https://dsmma.umn.edu/xiaotong-shen
This work is joint with Chunlin Li and Wei Pan at the University of Minnesota.
Xiaotong T. Shen is the John Black Johnston Distinguished Professor in the College of Liberal Arts at the University of Minnesota. His areas of interest include machine learning and data mining, high-dimensional analysis, graphical models, large margin methods, personalization, recommender systems, natural language processing and text mining, and nonconvex minimization. His current research effort is devoted to the further development of structured learning, collabrative learning, and scalable analysis. The targeted application areas are biomedical sciences, artificial intelligence, and engineering.
https://dsmma.umn.edu/xiaotong-shen
Explore Similar Events
-
Loading Similar Events...