Presented By: Center for Healthcare Engineering & Patient Safety (CHEPS)
Machine Learning and Causality: Building Efficient, Reliable Models for Decision-Making
Maggie Makar, Ph.D.
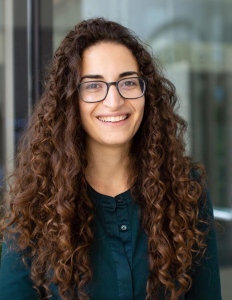
Increasingly practitioners are looking to ML-based causal and predictive models to provide personalized decision guidance. However, current techniques for causal inference typically rely on having access to large amounts of data, limiting their applicability to data-constrained settings such as healthcare where collection of data might be prohibitively costly. In addition, empirical evidence has shown that most predictive models are insufficiently robust with respect to shifts at test time. This talk presents novel techniques addressing both of these problems.
Much of the causal literature focuses on learning accurate individual treatment effects, which can be complex and hard to estimate from small samples. However, it is often sufficient for clinicians to have estimates of upper and lower bounds on the potential outcomes of decision alternatives to assess risks and benefits. I will show that in such cases we can improve sample efficiency by estimating simple functions that bound these outcomes instead of estimating their conditional expectations. I will present a novel algorithm that leverages these theoretical insights.
I will also present approaches to deal with distribution shifts. I will discuss how distribution shift arises when training models to predict contagious infections in the presence of asymptomatic carriers. I will present a causally-motivated regularization scheme that enables prediction of the true infection state with high accuracy even if the training data is collected under biased test administration.
Maggie Makar is the Presidential postdoctoral fellow at the University of Michigan, and a visiting research at Microsoft Research. Dr. Makar recieved her PhD in Computer Science at MIT. While at MIT, Maggie interned at Microsoft Research, and Google Brain. Prior to MIT, Maggie worked at Brigham and Women’s Hospital, studying end-of-life care. Her work has appeared in ICML, AAAI, JSM, the Journal of the American Medical Association (JAMA), Health Affairs, and Epidemiology among others. Maggie received a B.Sc. in Math and Economics from the University of Massachusetts, Amherst.
This seminar series is presented by the U-M Center for Healthcare Engineering and Patient Safety (CHEPS): Our mission is to improve the safety and quality of healthcare delivery through a multi-disciplinary, systems-engineering approach. For the Zoom link and password and to be added to the weekly e-mail for the series, please RSVP. For additional questions, contact CHEPSseminar@umich.edu. Photographs and video taken at this event may be used to promote CHEPS, College of Engineering, and the University.
Much of the causal literature focuses on learning accurate individual treatment effects, which can be complex and hard to estimate from small samples. However, it is often sufficient for clinicians to have estimates of upper and lower bounds on the potential outcomes of decision alternatives to assess risks and benefits. I will show that in such cases we can improve sample efficiency by estimating simple functions that bound these outcomes instead of estimating their conditional expectations. I will present a novel algorithm that leverages these theoretical insights.
I will also present approaches to deal with distribution shifts. I will discuss how distribution shift arises when training models to predict contagious infections in the presence of asymptomatic carriers. I will present a causally-motivated regularization scheme that enables prediction of the true infection state with high accuracy even if the training data is collected under biased test administration.
Maggie Makar is the Presidential postdoctoral fellow at the University of Michigan, and a visiting research at Microsoft Research. Dr. Makar recieved her PhD in Computer Science at MIT. While at MIT, Maggie interned at Microsoft Research, and Google Brain. Prior to MIT, Maggie worked at Brigham and Women’s Hospital, studying end-of-life care. Her work has appeared in ICML, AAAI, JSM, the Journal of the American Medical Association (JAMA), Health Affairs, and Epidemiology among others. Maggie received a B.Sc. in Math and Economics from the University of Massachusetts, Amherst.
This seminar series is presented by the U-M Center for Healthcare Engineering and Patient Safety (CHEPS): Our mission is to improve the safety and quality of healthcare delivery through a multi-disciplinary, systems-engineering approach. For the Zoom link and password and to be added to the weekly e-mail for the series, please RSVP. For additional questions, contact CHEPSseminar@umich.edu. Photographs and video taken at this event may be used to promote CHEPS, College of Engineering, and the University.
Explore Similar Events
-
Loading Similar Events...