Presented By: Department of Statistics
Dissertation Defense: Dry runs and PWRD aggregation: Two new methods for extracting power from careful observation of a field experiment's context
Timothy Lycurgus, PhD Candidate, University of Michigan.
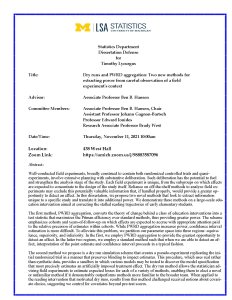
Abstract:
Well-conducted field experiments, broadly construed to contain both randomized controlled trials and quasi- experiments, involve extensive planning with substantive deliberation. Such deliberation has the potential to fuel and strengthen the analysis stage of the study. Each field experiment is unique, from the subgroups on which effects are expected to concentrate to the design of the study itself. Reliance on off the-shelf methods to analyze field experiments may exclude this potentially valuable information that, if handled properly, would provide a greater opportunity to detect an effect. In this dissertation, we propose two novel methods that look to extract information unique to a specific study and translate it into additional power. We demonstrate these methods on a large-scale education intervention aimed at correcting the stalled reading trajectories of early elementary students.
The first method, PWRD aggregation, converts the theory of change behind a class of education interventions into a test statistic that maximizes the Pitman efficiency over standard methods, thus providing greater power. The scheme emphasizes cohorts and years-of-follow-up on which effects are expected to accrue with appropriate attention paid to the relative precision of estimates within cohorts. While PWRD aggregation increases power, confidence interval estimation is more difficult. To alleviate this problem, we partition our parameter space into three regions: equivalence, superiority, and inferiority. In the first, we employ PWRD aggregation to provide the greatest opportunity to detect an effect. In the latter two regions, we employ a standard method such that when we are able to detect an effect, interpretation of the point estimate and confidence interval proceeds in a typical fashion.
The second method we propose is a dry run simulation scheme that creates a pseudo-experiment replicating the initial randomized trial in a manner that preserves blinding to impact estimates. This procedure, which uses real rather than synthetic data, provides a sandbox in which various models may be tested to discover the model specification that most precisely estimates an artificially imposed treatment effect. The dry run method allows the statistician advising field experiments to estimate expected losses for each of a variety of methods, enabling them to elect a novel or unfamiliar method if it demonstrably outperforms methods more familiar to the broader team. When applied to the reading intervention that motivated dry runs, results from this method challenged received notions about covariate choice, suggesting we control for covariates beyond pre-test scores.
Well-conducted field experiments, broadly construed to contain both randomized controlled trials and quasi- experiments, involve extensive planning with substantive deliberation. Such deliberation has the potential to fuel and strengthen the analysis stage of the study. Each field experiment is unique, from the subgroups on which effects are expected to concentrate to the design of the study itself. Reliance on off the-shelf methods to analyze field experiments may exclude this potentially valuable information that, if handled properly, would provide a greater opportunity to detect an effect. In this dissertation, we propose two novel methods that look to extract information unique to a specific study and translate it into additional power. We demonstrate these methods on a large-scale education intervention aimed at correcting the stalled reading trajectories of early elementary students.
The first method, PWRD aggregation, converts the theory of change behind a class of education interventions into a test statistic that maximizes the Pitman efficiency over standard methods, thus providing greater power. The scheme emphasizes cohorts and years-of-follow-up on which effects are expected to accrue with appropriate attention paid to the relative precision of estimates within cohorts. While PWRD aggregation increases power, confidence interval estimation is more difficult. To alleviate this problem, we partition our parameter space into three regions: equivalence, superiority, and inferiority. In the first, we employ PWRD aggregation to provide the greatest opportunity to detect an effect. In the latter two regions, we employ a standard method such that when we are able to detect an effect, interpretation of the point estimate and confidence interval proceeds in a typical fashion.
The second method we propose is a dry run simulation scheme that creates a pseudo-experiment replicating the initial randomized trial in a manner that preserves blinding to impact estimates. This procedure, which uses real rather than synthetic data, provides a sandbox in which various models may be tested to discover the model specification that most precisely estimates an artificially imposed treatment effect. The dry run method allows the statistician advising field experiments to estimate expected losses for each of a variety of methods, enabling them to elect a novel or unfamiliar method if it demonstrably outperforms methods more familiar to the broader team. When applied to the reading intervention that motivated dry runs, results from this method challenged received notions about covariate choice, suggesting we control for covariates beyond pre-test scores.
Explore Similar Events
-
Loading Similar Events...