Presented By: U-M Industrial & Operations Engineering
IOE JOB TALK PRACTICE: "Multistage Distributionally Robust Mixed-Integer Programming with Decision-Dependent Moment-Based Ambiguity Sets" - Xian Yu
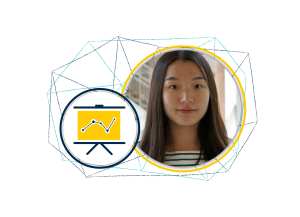
Seminar Abstract: We study multistage distributionally robust mixed-integer programs under endogenous uncertainty, where the probability distribution of stage-wise uncertainty depends on the decisions made in previous stages. We first consider two ambiguity sets defined by decision- dependent bounds on the first and second moments of uncertain parameters and by mean and covariance matrix that exactly match decision-dependent empirical ones, respectively. For both sets, we show that the subproblem in each stage can be recast as a mixed-integer linear program. Moreover, we extend the general moment-based ambiguity set in Delage and Ye (2010) to the multistage decision-dependent setting and derive mixed-integer semidefinite programming (MISDP) reformulations of stage-wise subproblems. We obtain lower and upper bounds of the optimal objective value of the multistage MISDPs. We deploy the Stochastic Dual Dynamic integer Programming (SDDiP) method for solving the problem with risk-neutral or risk- averse objective functions, and conduct numerical studies on multistage facility-location instances having diverse sizes under different parameter and uncertainty settings. Our results show that the SDDiP quickly finds optimal solutions for moderate-sized instances under the first two ambiguity sets, and also finds good approximate bounds for the multistage MISDPs derived under the third ambiguity set. We also demonstrate the efficacy of incorporating decision- dependent distributional ambiguity in multistage decision-making processes. This general decision-making framework can be applied to a broad spectrum of applications, such as shared mobility, supply chain management, and finance. In the future, I look forward to continuing this line of research and combining risk management with reinforcement learning to enhance risk- aware decisions.
Presenter Bio: Xian Yu is currently a Ph.D. candidate from Department of Industrial and Operations Engineering at the University of Michigan. Her research interest mainly lies in sequential decision-making and optimization under uncertainty, with applications in transportation, healthcare and supply chain management. Her dissertation aims to bridge theories in stochastic programming, distributionally robust optimization, integer programming and dynamic programming, as well as propose efficient algorithms for solving large-scale real-world problems under uncertainty. Xian has been awarded IOE Katta Murty Prize for the Best Research Paper on Optimization in 2021, and Michigan Institute for Computational Discovery and Engineering Fellowship in 2019.
Presenter Bio: Xian Yu is currently a Ph.D. candidate from Department of Industrial and Operations Engineering at the University of Michigan. Her research interest mainly lies in sequential decision-making and optimization under uncertainty, with applications in transportation, healthcare and supply chain management. Her dissertation aims to bridge theories in stochastic programming, distributionally robust optimization, integer programming and dynamic programming, as well as propose efficient algorithms for solving large-scale real-world problems under uncertainty. Xian has been awarded IOE Katta Murty Prize for the Best Research Paper on Optimization in 2021, and Michigan Institute for Computational Discovery and Engineering Fellowship in 2019.
Explore Similar Events
-
Loading Similar Events...