Presented By: Michigan Robotics
Visual Methods Towards Autonomous Underwater Manipulation
PhD Defense, Gideon Billings
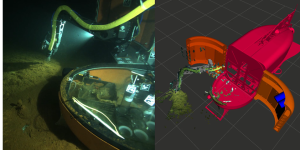
Chair: Matthew Johnson Roberson
Extra-terrestrial ocean worlds like Europa offer tantalizing targets in the search for extant life beyond the confines of Earth's atmosphere. However, reaching and exploring the underwater environments of these alien worlds is a task with immense challenges. Unlike terrestrial based missions, the exploration of ocean worlds necessitates robots which are capable of fully automated operation. These robots must rely on local sensors to interpret the scene, plan their motions, and complete their mission tasks. Manipulation tasks, such as sample collection, are particularly challenging in underwater environments, where the manipulation platform is mobile, and the environment is unstructured.
This dissertation addresses some of the challenges in visual scene understanding to support autonomous manipulation with underwater vehicle manipulator systems (UVMSs). The developed visual methods are demonstrated with a lightweight vision system, composed of a vehicle mounted stereo pair and a manipulator wrist mounted fisheye camera, that can be easily integrated on existing UVMSs. While the stereo camera primarily supports 3D reconstruction of the manipulator working area, the wrist mounted camera enables dynamic viewpoint acquisition for detecting objects, such as tools, and extending the scene reconstruction beyond the fixed stereo view. An objective of this dissertation was also to apply deep learning based visual methods to the underwater domain. While deep learning has greatly advanced the state-of-the-art in terrestrial based visual methods across diverse applications, the challenges of accessing the underwater environment and collecting underwater datasets for training these methods has hindered progress in advancing visual methods for underwater applications.
Following is an overview of the contributions made by this dissertation. The first contribution is a novel deep learning method for object detection and pose estimation from monocular images. The second contribution is a general framework for adapting monocular image-based pose estimation networks to work on full fisheye or omni-directional images with minimal modification to the network architecture. The third contribution is a visual SLAM method designed for UVMSs that fuses features from both the wrist mounted fisheye camera and the vehicle mounted stereo pair into the same map, where the map scale is constrained by the stereo features, and the wrist camera can actively extend the map beyond the limited stereo view. The fourth contribution is an open-source tool to aid the design of underwater camera and lighting systems. The fifth contribution is an autonomy framework for UVMS manipulator control and the vision system that was used throughout this dissertation work, along with experimental results from field trials in natural deep ocean environments, including an active submarine volcano in the Mediterranean basin. This chapter also describes the collection and processing of underwater datasets, captured with our vision system in these natural deep ocean environments. These datasets supported the development of the visual methods contributed by this dissertation.
Extra-terrestrial ocean worlds like Europa offer tantalizing targets in the search for extant life beyond the confines of Earth's atmosphere. However, reaching and exploring the underwater environments of these alien worlds is a task with immense challenges. Unlike terrestrial based missions, the exploration of ocean worlds necessitates robots which are capable of fully automated operation. These robots must rely on local sensors to interpret the scene, plan their motions, and complete their mission tasks. Manipulation tasks, such as sample collection, are particularly challenging in underwater environments, where the manipulation platform is mobile, and the environment is unstructured.
This dissertation addresses some of the challenges in visual scene understanding to support autonomous manipulation with underwater vehicle manipulator systems (UVMSs). The developed visual methods are demonstrated with a lightweight vision system, composed of a vehicle mounted stereo pair and a manipulator wrist mounted fisheye camera, that can be easily integrated on existing UVMSs. While the stereo camera primarily supports 3D reconstruction of the manipulator working area, the wrist mounted camera enables dynamic viewpoint acquisition for detecting objects, such as tools, and extending the scene reconstruction beyond the fixed stereo view. An objective of this dissertation was also to apply deep learning based visual methods to the underwater domain. While deep learning has greatly advanced the state-of-the-art in terrestrial based visual methods across diverse applications, the challenges of accessing the underwater environment and collecting underwater datasets for training these methods has hindered progress in advancing visual methods for underwater applications.
Following is an overview of the contributions made by this dissertation. The first contribution is a novel deep learning method for object detection and pose estimation from monocular images. The second contribution is a general framework for adapting monocular image-based pose estimation networks to work on full fisheye or omni-directional images with minimal modification to the network architecture. The third contribution is a visual SLAM method designed for UVMSs that fuses features from both the wrist mounted fisheye camera and the vehicle mounted stereo pair into the same map, where the map scale is constrained by the stereo features, and the wrist camera can actively extend the map beyond the limited stereo view. The fourth contribution is an open-source tool to aid the design of underwater camera and lighting systems. The fifth contribution is an autonomy framework for UVMS manipulator control and the vision system that was used throughout this dissertation work, along with experimental results from field trials in natural deep ocean environments, including an active submarine volcano in the Mediterranean basin. This chapter also describes the collection and processing of underwater datasets, captured with our vision system in these natural deep ocean environments. These datasets supported the development of the visual methods contributed by this dissertation.
Explore Similar Events
-
Loading Similar Events...