Presented By: Michigan Robotics
Tackling Limited Sensing Capabilities for Autonomous Driving
PhD Defense, Ming-Yuan Yu
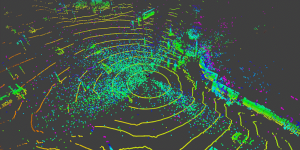
Chair: Matthew Johnson-Roberson
Abstract:
Limited sensing capabilities pose challenges to autonomous vehicles. Common limitations can result from a limited sensory range, occlusions, or adverse weather conditions, and hinder the development of both general and safe autonomy in real-world environments. In the pursuit of safe autonomy under imperfect measurements, an autonomous vehicle must assess the risk to take safe actions, and actively gather informative data to reduce the uncertainty. This dissertation introduces efficient algorithms for risk assessment and noise removal, specifically with LiDAR, to aid the safe navigation of autonomous vehicles.
First, a probabilistic risk assessment algorithm is proposed, which utilizes scene geometry to quantify the risk imposed by occluded oncoming traffic. Simulations show that the quantified risk can then be used as an objective function of a subsequent planning algorithm to improve both safety and ride comfort at real-world urban intersections. Second, the efficiency of the aforementioned algorithm is further enhanced by leveraging reachability analysis to constrain its sensory resources to only regions with potential collisions. This leads to an algorithm that not only quantifies the risk more efficiently, but also localizes the risk-inducing traffic participants in an environment. As a result, unlike the previous approach, the vehicle no longer needs to put equal attention to the entire scene and could focus only on essential information in collision avoidance. Lastly, a real-time de-noising algorithm is proposed to remove noise from LiDAR point clouds corrupted by snow. This reduces uncertainty from adverse weather conditions. The proposed de-noising network outperforms state-of-the-art methods while also running orders of magnitude faster. The de-noised point clouds also show positive impact to off-the-shelf SLAM algorithms, resulting in maps nearly free of noise while preserving salient information of the scene.
These algorithms are efficient and make safe autonomy feasible in the near future, even under imperfect LiDAR measurements.
Abstract:
Limited sensing capabilities pose challenges to autonomous vehicles. Common limitations can result from a limited sensory range, occlusions, or adverse weather conditions, and hinder the development of both general and safe autonomy in real-world environments. In the pursuit of safe autonomy under imperfect measurements, an autonomous vehicle must assess the risk to take safe actions, and actively gather informative data to reduce the uncertainty. This dissertation introduces efficient algorithms for risk assessment and noise removal, specifically with LiDAR, to aid the safe navigation of autonomous vehicles.
First, a probabilistic risk assessment algorithm is proposed, which utilizes scene geometry to quantify the risk imposed by occluded oncoming traffic. Simulations show that the quantified risk can then be used as an objective function of a subsequent planning algorithm to improve both safety and ride comfort at real-world urban intersections. Second, the efficiency of the aforementioned algorithm is further enhanced by leveraging reachability analysis to constrain its sensory resources to only regions with potential collisions. This leads to an algorithm that not only quantifies the risk more efficiently, but also localizes the risk-inducing traffic participants in an environment. As a result, unlike the previous approach, the vehicle no longer needs to put equal attention to the entire scene and could focus only on essential information in collision avoidance. Lastly, a real-time de-noising algorithm is proposed to remove noise from LiDAR point clouds corrupted by snow. This reduces uncertainty from adverse weather conditions. The proposed de-noising network outperforms state-of-the-art methods while also running orders of magnitude faster. The de-noised point clouds also show positive impact to off-the-shelf SLAM algorithms, resulting in maps nearly free of noise while preserving salient information of the scene.
These algorithms are efficient and make safe autonomy feasible in the near future, even under imperfect LiDAR measurements.
Explore Similar Events
-
Loading Similar Events...