Presented By: Michigan Robotics
Semantic-Aware Robotic Mapping in Unknown, Loosely Structured Environments
Phd Defense, Lu Gan
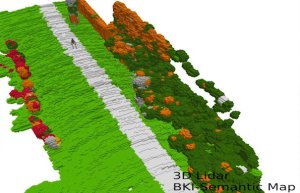
Chair: Ryan Eustice
Abstract:
Robotic mapping is the problem of inferring a representation of a robot’s surroundings using noisy measurements as it navigates through an environment. As robotic systems move toward more challenging behaviors in more complex scenarios, such systems require richer maps so that the robot understands the significance of the scene and objects within.
This dissertation focuses on semantic-aware robotic mapping in unknown, loosely structured environments. The first contribution is a Bayesian kernel inference semantic mapping framework that formulates a unified probabilistic model for occupancy and semantics, and provides a closed-form solution for scalable dense semantic mapping. This framework significantly reduces the computational complexity of learning-based continuous semantic mapping and achieves high accuracy in the meantime. Next, a novel and flexible multi-task multi-layer Bayesian mapping framework is proposed to provide even richer environmental information. A two-layer robotic map of semantics and traversability is built as a strong example. Moreover, it is readily extendable to include more layers according to needs. Both mapping algorithms were verified using publicly available datasets or through experimental results on a Cassie-series bipedal robot. Finally, instead of modeling the terrain traversability using metrics defined by domain knowledge, an energy-based deep inverse reinforcement learning method is developed to learn the traversability from demonstrations. The proposed method considers robot proprioception and can learn reward maps that lead to more energy-efficient future trajectories. Experiments are conducted using a dataset collected by a Mini-Cheetah robot in different environments.
Abstract:
Robotic mapping is the problem of inferring a representation of a robot’s surroundings using noisy measurements as it navigates through an environment. As robotic systems move toward more challenging behaviors in more complex scenarios, such systems require richer maps so that the robot understands the significance of the scene and objects within.
This dissertation focuses on semantic-aware robotic mapping in unknown, loosely structured environments. The first contribution is a Bayesian kernel inference semantic mapping framework that formulates a unified probabilistic model for occupancy and semantics, and provides a closed-form solution for scalable dense semantic mapping. This framework significantly reduces the computational complexity of learning-based continuous semantic mapping and achieves high accuracy in the meantime. Next, a novel and flexible multi-task multi-layer Bayesian mapping framework is proposed to provide even richer environmental information. A two-layer robotic map of semantics and traversability is built as a strong example. Moreover, it is readily extendable to include more layers according to needs. Both mapping algorithms were verified using publicly available datasets or through experimental results on a Cassie-series bipedal robot. Finally, instead of modeling the terrain traversability using metrics defined by domain knowledge, an energy-based deep inverse reinforcement learning method is developed to learn the traversability from demonstrations. The proposed method considers robot proprioception and can learn reward maps that lead to more energy-efficient future trajectories. Experiments are conducted using a dataset collected by a Mini-Cheetah robot in different environments.
Explore Similar Events
-
Loading Similar Events...