Presented By: Department of Psychology
CCN Forum:
Cody Cao and Logan Walls, Graduate Students, Cognition and Cognitive Neuroscience
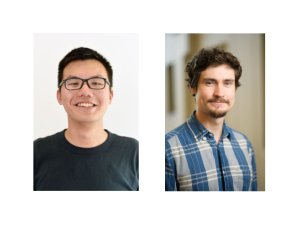
Cody
Title:
Listeners extract spectral and temporal information from the mouth during naturalistic audiovisual speech
Abstract:
Seeing a speaker’s face helps speech perception. But, what features of the face convey meaningful speech information? Although visual signals from the mouth have been shown to restore auditory speech information, it remains possible that statistical features including temporal and spectral information can be extracted from other regions of the face. Here, we test whether viewing the mouth is sufficient for restoring spectral and temporal speech information. Across three different experiments, using eye-tracking, partial occlusion of faces, and extraction of features from the face using a deep learning toolkit, we tested whether spectral and temporal speech information is recovered from different regions of the face. Preliminary results across all studies demonstrate that viewing the mouth is necessary and sufficient for the extraction and use of lipreading, temporal and spectral speech information.
Logan
Title:
Cognitive & Linguistic Biases of Transformer Language Models
Abstract:
Recent neural-net language models such as GPT-2/3 have achieved unprecedented advances in tasks ranging from machine translation to text summarization. These models are of growing interest in psycholinguistics, and cognitive science more broadly, in part because the models’ learned representations provide the basis for quantitative predictions of human data such as gaze durations in eye-tracking reading studies, and the models’ internal processing of such models is interpretable in terms of interference-based memory theories. We outline a general method to probe the nature of the inductive biases in neural-net language models (biases that are not simply reflections of the big-data on which they are trained), asking questions about how these biases may give shape to attested properties of human language, and illustrate the method with a preliminary study of GPT-2's biases for syntactic dependency length –which has played important roles in sentence processing research and in cross-linguistic typological studies.
Title:
Listeners extract spectral and temporal information from the mouth during naturalistic audiovisual speech
Abstract:
Seeing a speaker’s face helps speech perception. But, what features of the face convey meaningful speech information? Although visual signals from the mouth have been shown to restore auditory speech information, it remains possible that statistical features including temporal and spectral information can be extracted from other regions of the face. Here, we test whether viewing the mouth is sufficient for restoring spectral and temporal speech information. Across three different experiments, using eye-tracking, partial occlusion of faces, and extraction of features from the face using a deep learning toolkit, we tested whether spectral and temporal speech information is recovered from different regions of the face. Preliminary results across all studies demonstrate that viewing the mouth is necessary and sufficient for the extraction and use of lipreading, temporal and spectral speech information.
Logan
Title:
Cognitive & Linguistic Biases of Transformer Language Models
Abstract:
Recent neural-net language models such as GPT-2/3 have achieved unprecedented advances in tasks ranging from machine translation to text summarization. These models are of growing interest in psycholinguistics, and cognitive science more broadly, in part because the models’ learned representations provide the basis for quantitative predictions of human data such as gaze durations in eye-tracking reading studies, and the models’ internal processing of such models is interpretable in terms of interference-based memory theories. We outline a general method to probe the nature of the inductive biases in neural-net language models (biases that are not simply reflections of the big-data on which they are trained), asking questions about how these biases may give shape to attested properties of human language, and illustrate the method with a preliminary study of GPT-2's biases for syntactic dependency length –which has played important roles in sentence processing research and in cross-linguistic typological studies.
Livestream Information
LivestreamFebruary 4, 2022 (Friday) 2:00pm
Joining Information Not Yet Available
Explore Similar Events
-
Loading Similar Events...