Presented By: Department of Statistics
Statistics Department Seminar Series: Jeff Regier, Assistant Professor, Department of Statistics, University of Michigan
"Modern Variational Inference for Interpreting Astronomical Images"
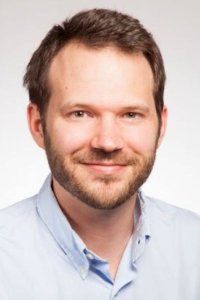
Abstract: Bayesian statistics provides an elegant formalism for interpreting astronomical images: scientifically interesting but unknown properties of imaged stars and galaxies are treated as latent random variables and pixel intensities are treated as observed random variables. It is challenging, however, to efficiently infer the posterior distribution for realistic Bayesian models of astronomical images. In this talk, I present the Bayesian Light Source Separator (BLISS), which overcomes this challenge through the judicious use of modern variational inference techniques. BLISS embeds a flexible galaxy model that is encoded by a neural network within an interpretable generative model of astronomical images, and employs neural networks, stochastic optimization, and a novel variational bound to facilitate posterior inference. In comparison to a recently published method based on MCMC for detecting astronomical objects in crowded fields, BLISS performs posterior inference 10,000 times faster while achieving substantially higher accuracy.
https://regier.stat.lsa.umich.edu/
https://regier.stat.lsa.umich.edu/